- ASR technology utilises machine learning and signal processing to convert human speech into digital signals for recognition by computers, enabling a wide range of applications from smart homes to healthcare and education.
- Challenges faced by ASR include the complexity of human speech, noise interference, context considerations, data volume and quality, algorithm requirements, and privacy concerns regarding data processing and storage.
- Future directions for ASR development include multilingual speech recognition, reinforcement learning algorithms, multimodal fusion, edge computing, and human-computer interaction enhancements with a focus on privacy protection and security.
In the past, people needed to use input devices such as keyboards to give instructions to computers, a method that required cumbersome input operations and time. However, with the continuous development and refinement of Automatic speech recognition (ASR) technology, people can now interact directly with computers through speech, achieving a more natural and convenient human-computer interaction method. Through ASR technology, individuals can easily use speech to open applications, search for information, initiate calls, and perform other tasks, no longer relying on cumbersome input operations. This makes human-computer interaction more intelligent and efficient.
Introduction to ASR
ASR technology is a technique based on machine learning and signal processing, among other technologies. It converts human speech into digital signals that computers can process, recognising them as corresponding text, commands, or operational instructions.
ASR technology typically consists of three main parts: signal processing, speech recognition, and result processing. Signal processing involves transforming raw audio signals into a form suitable for speech recognition, such as noise reduction and speech enhancement. Speech recognition entails converting the processed audio signal into text form recognisable by computers, often achieved through word or phoneme recognition. Result processing involves converting the text recognised by the computer into readable text output.
Also read: Reebok launches AI-powered fashion experience on Instagram
Applications scenarios of ASR
ASR technology finds wide application across various domains, enabling more efficient, convenient, and intelligent ways of working and living:
Smart homes
Users can control smart home devices through voice commands, such as turning on/off lights or adjusting temperature.
Intelligent customer service
Firms utilise ASR for self-service and intelligent customer support, including features like automated call answering, voice navigation, and intelligent FAQs.
Smart speakers
ASR is integral to smart speakers, allowing users to control music playback, make calls, send messages, and more via voice commands.
Speech recognition assistants
ASR facilitates speech input, such as voice input keyboards and voice memo apps on smartphones.
Voice search
Users can quickly search for information using voice commands through voice search engines.
Autonomous driving
ASR technology is extensively used in autonomous vehicles, enabling voice commands for vehicle control and operation.
Healthcare
Doctors and nurses can input patient information through speech, avoiding tedious recording processes. ASR can also automatically transcribe conversations between doctors and patients, aiding doctors in better understanding patient conditions.
Education
Students can practice oral expression using ASR technology and receive real-time feedback and suggestions. Teachers can utilise ASR to record classroom discussions and help students better understand course content.
Also read: Remini’s clay filter: What makes this app so popular in China?
Challenges faced by ASR
Although ASR technology has made significant advancements in the field of human-computer interaction, it still faces a series of challenges, such as how to ensure accuracy, stability, and timeliness. Several aspects have a crucial impact on the performance of ASR:
Variety of speech
Human speech is highly complex and diverse, including various accents, dialects, intonations, speech rates, pronunciations, etc. This diversity poses significant challenges for the development and application of ASR technology as it needs to overcome these variations and be able to recognise various forms of speech.
Noise and interference in speech
Speech signals are often accompanied by various noises and interferences, such as background noise, cross-talk, coughing, etc. These noises and interferences severely affect the performance and accuracy of ASR technology.
Context and context of language
Speech recognition needs to consider the context and context of language, such as grammar, sentence structure, semantics, lexical collocations, etc. These factors are crucial for the accuracy and reliability of speech recognition but also present challenges for ASR technology.
Volume and quality of data
ASR technology requires a large amount of training data to improve its accuracy and performance. However, the quality and quantity of training data can significantly affect the performance of ASR technology, making acquiring a sufficient amount of high-quality data another challenge.
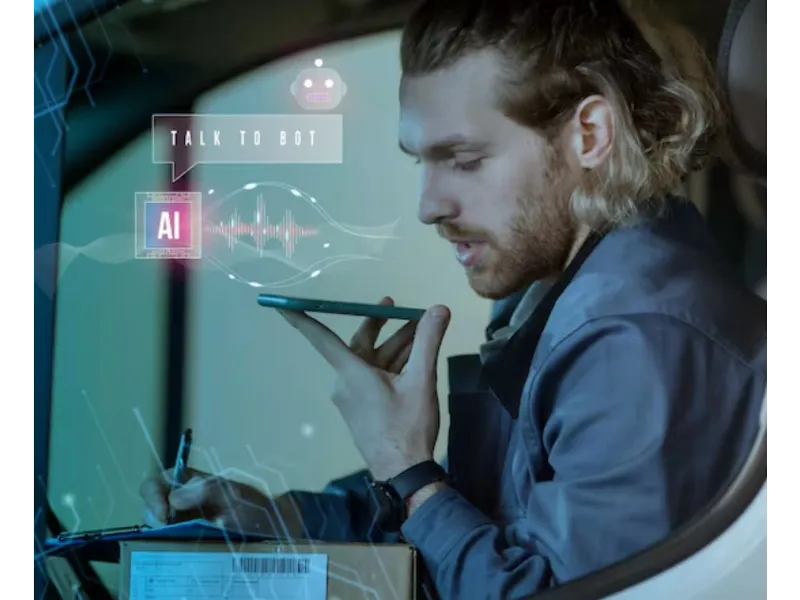
Speech recognition algorithms
Currently, ASR technology mainly uses statistical models and deep learning algorithms, which require substantial computational resources and support from technical personnel. Additionally, continuous improvement and optimisation are needed to meet the requirements of different application scenarios.
Personal privacy and data security
ASR technology requires data processing and storage through cloud services, raising concerns about personal privacy and data security. Therefore, protecting user privacy and data security are essential issues for the development of ASR technology.
Development directions of ASR
The future development directions of ASR technology face numerous challenges, but with continuous technological innovation and practical applications, along with the ongoing development in fields like artificial intelligence and natural language processing, ASR technology is poised for wider application and advancement.
In the future, the development directions of ASR technology may include the following aspects:
Multilingual speech recognition
With globalisation accelerating and multilingual environments becoming more prevalent, multilingual speech recognition technology will become increasingly important. Future ASR technology needs to support recognition in multiple languages and consider the speech characteristics and differences between different languages. Additionally, research into models that can encode multiple languages is underway, aiming to develop models capable of handling various languages instead of building separate models for each language.
Reinforcement learning and deep reinforcement learning
Traditional ASR technology primarily relies on statistical models and deep learning algorithms, which still face challenges such as requiring large amounts of annotated data and computational resources. In the future, ASR technology may utilise algorithms like reinforcement learning to enhance efficiency and accuracy in specific scenarios, such as dialogue systems and natural language processing tasks.
Multimodal fusion
While speech recognition technology typically relies solely on speech signals, future ASR technology may integrate information from other modalities such as video, images, and text to improve performance and accuracy. Visual speech recognition or joint models for speech and text are current research hotspots in this area.
Edge computing and human-computer interaction
Future ASR technology may focus more on edge computing and human-computer interaction to achieve more efficient and intelligent speech recognition and interaction experiences. Edge computing involves processing data at the network edge (such as user devices or network nodes close to users), reducing latency, and protecting user privacy. Human-computer interaction focuses on the study of how people and computers communicate and interact.
Privacy protection and security
With increasing attention to user privacy and data security, future ASR technology needs to better protect user privacy and data security, for example, by using more secure encryption techniques and decentralised storage. Additionally, performing ASR on devices (rather than in the cloud) is a trend that can better protect user privacy.