- Data mining technology enhances market analysis and customer segmentation in retail, as well as facilitating personalised treatment plans and predictive healthcare analytics.
- Data mining technology empowers financial institutions to effectively manage risks, optimise investment strategies, enhance credit scoring accuracy, and identify market trends, thereby improving decision-making and financial performance.
- Data mining technology enhances manufacturing by optimising production processes, improving supply chain agility, and ensuring product quality, leading to increased efficiency, reduced risks, and higher customer satisfaction.
Data mining helps in finding patterns from the data sets used to create predictive models and then applying predictive algorithms to these predictive models to make accurate predictions. Data mining’s ability to detect patterns and relationships from data can help organisations make better decisions.
Also read: IoT data integration: Unlocking insights for a smarter future
Retail
In the business world, data mining is widely used for market analysis and customer segmentation. Through data mining technology, enterprises can deeply analyse consumers’ behaviours, preferences and purchasing habits, so as to better understand customers’ needs and conduct accurate market positioning and customer segmentation. For example, retailers can analyse shopping basket data to find out which items are often purchased together, so as to conduct joint promotions and increase sales.
Data mining plays an important role in customer relationship management. By analysing customers’ transaction records, interaction records and feedback information, companies can identify high-value customers, churned customers and potential customers, and formulate marketing strategies accordingly. For example, through predictive modelling, companies can identify customers who are likely to be lost and take early retention measures to improve customer loyalty and satisfaction.
Data mining technology can help companies make sales forecasts. By analysing historical sales data, market trends and external factors, enterprises can build forecasting models to predict future sales, so as to rationally arrange production plans and inventory management, reduce inventory backlogs and out-of-stock risks, and improve the efficiency of enterprise operations.
For example, Amazon analyses users’ browsing history, purchase records, shopping cart data and search history through data mining technology to build a user interest model, and then provides personalised product recommendations for each user.
Also read: A look at cloud data management
Healthcare
The healthcare industry collects a great deal of data through health documents in the form of wearable devices or electronic health records (EHRs). Data mining techniques can help gain insights from this data to provide optimal treatment and better service to patients.
Data mining can compare the efficacy of various drugs in treating specific diseases in different age groups. Thus, data mining can help in determining the best standard drug for a disease.
Personalised medicine is the development of a personalised treatment plan based on an individual’s genes, environment and lifestyle. Data mining technology can help healthcare providers analyse a patient’s genetic data and medical records to identify disease-related genetic variants and provide personalised treatment recommendations. For example, cancer patients can use genetic testing to identify the most suitable targeted therapy drugs and improve treatment outcomes
For example, North Carolina State University and the Mayo Clinic collaborated to develop a model for predicting heart attack risk. The model predicts a patient’s risk of having a heart attack in the next few years by analysing the patient’s electronic health records (EHRs), including data on age, gender, blood pressure, cholesterol levels, lifestyle and more.
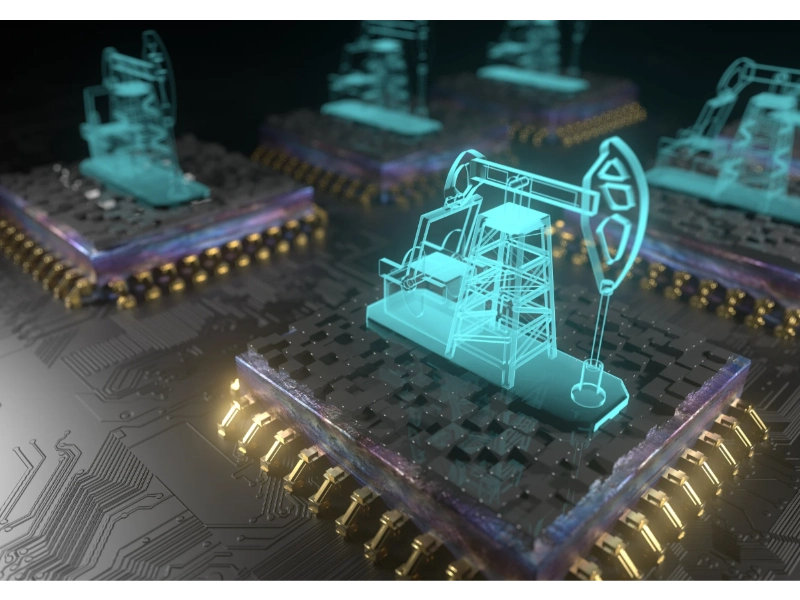
Financial
Data mining technology can help financial institutions identify and assess various risks, such as credit risk, market risk and operational risk.
By analysing customers’ financial data, transaction data and market data, financial institutions can build risk prediction models, identify potential risks promptly and take appropriate risk control measures. For example, by analysing credit card transaction data, high-risk customers can be identified, and bad debt losses can be prevented.
Data mining technology can be used to analyse historical price data, financial statement data and market indicator data of stocks, bonds and other financial products so that investors can assess investment risks and returns and formulate optimal investment portfolio strategies. For example, through machine learning algorithms, it is possible to predict stock price movements, optimise investment decisions and improve investment returns.
By analysing a customer’s credit history, financial status and repayment behaviour, financial institutions can build a credit scoring model to assess the customer’s credit risk and reasonably determine the loan amount and interest rate. For example, by analysing a borrower’s historical repayment records, it is possible to predict his or her future default risk and improve the accuracy and efficiency of loan approval.
For example, FICO‘s credit scoring model makes use of information such as a customer’s credit history, repayment record and debt level to assess a customer’s credit risk and provide credit scoring services to banks and credit card companies.
Data mining technology can help financial institutions conduct market trend analysis. By analysing a large amount of market data, economic data and news data, financial institutions can identify market trends and investment opportunities, formulate corresponding investment strategies and reduce investment risks.
Manufacturing
By analysing production data, equipment data and quality data, enterprises can identify bottlenecks and problems in the production process, propose improvement measures, and improve production efficiency and product quality.
For example, Siemens uses IoT sensors and data collection systems to monitor all aspects of the production line in real time and collect a large amount of production data. Through data mining and analysis, Siemens is able to identify production bottlenecks, optimise production scheduling and improve production efficiency.
Data mining technology can improve the agility and responsiveness of the supply chain by analysing supply chain data, inventory data and market demand data, which allows companies to rationally arrange procurement, production and logistics.
For example, Cisco collects and analyses a large amount of data in its supply chain, including supplier performance, inventory levels, and shipping times. Through data mining and machine learning algorithms, Cisco can predict potential risks in the supply chain, such as supplier delays and inventory shortages.
Data mining has important applications in product quality control. By analysing production data, quality inspection data and customer feedback data, enterprises can identify key factors affecting product quality, propose improvement measures, and improve product quality and customer satisfaction.
For example, GE collects a large amount of operational data, including key parameters such as temperature, pressure, and vibration, through sensors installed on equipment. Using data mining technology, GE can analyse this data in real-time to detect potential failures and performance degradation trends.