- Anomaly detection identifies unusual patterns in network traffic to prevent security breaches.
- Advanced technologies like AI and machine learning enhance anomaly detection capabilities, protecting networks in real time.
Anomaly detection is a crucial component in network security, designed to identify unusual behavior or patterns that may indicate security threats, such as unauthorized access, malware, or data breaches. By monitoring normal network behavior and flagging deviations, anomaly detection systems act as an early warning mechanism, preventing minor issues from escalating into major cyberattacks.
Modern IT infrastructure relies on advanced anomaly detection systems powered by AI, machine learning, and statistical models to ensure robust network protection. As cyberattacks become more sophisticated, organizations must embrace anomaly detection tools to secure their systems, protect sensitive data, and maintain business continuity.
How anomaly detection works
Anomaly detection operates by establishing a baseline for “normal” network behavior. By continuously analyzing incoming traffic and user activities, the system identifies deviations that may signal potential threats.
Key steps in anomaly detection include:
- Data collection: Monitoring network traffic, logs, and system performance.
- Baseline behavior analysis: Using historical data to define normal patterns.
- Anomaly identification: Detecting deviations based on predefined thresholds or dynamic models.
- Alert generation: Flagging unusual activities for further investigation.
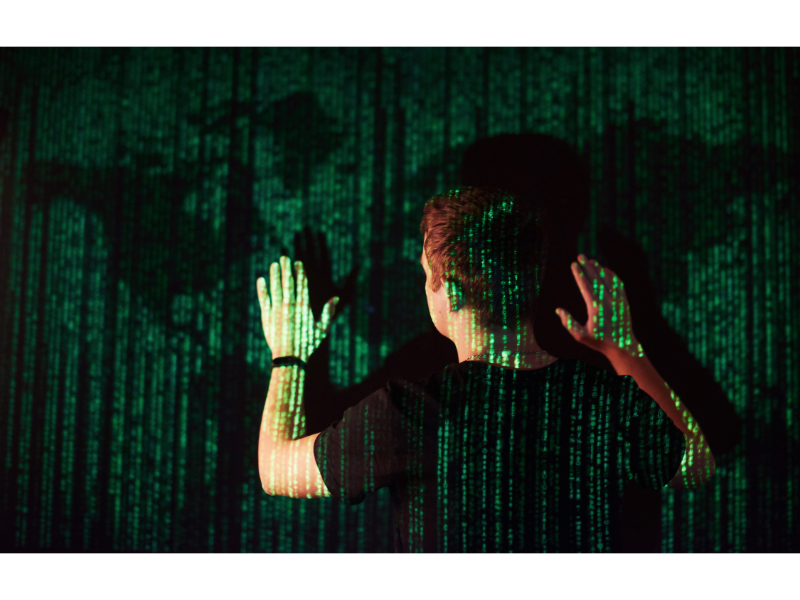
Also read: The importance of anomaly detection in data analysis
Types of anomaly detection
1. Statistical anomaly detection
This approach uses statistical methods to identify patterns that fall outside the expected range.
- Example: Identifying a sudden spike in traffic volume during non-business hours.
- Pros: Simple and effective for predictable environments.
- Cons: May generate false positives in dynamic networks.
2. Rule-based anomaly detection
This method relies on pre-defined rules to determine normal behavior.
- Example: Blocking logins from unknown IP addresses.
- Pros: Easy to implement and understand.
- Cons: Rules need constant updating to remain effective.
3. Machine learning-based anomaly detection
Machine learning algorithms analyze large datasets to detect anomalies and adapt to changing behaviors.
- Example: Using AI to identify unusual login times or access patterns.
- Pros: Highly effective for dynamic, large-scale networks.
- Cons: Requires significant computational resources and training data.
Why anomaly detection is critical for network security
- Early threat detection
Anomaly detection systems can identify potential threats before they cause damage. By flagging unusual activities, they allow organizations to respond quickly to security breaches. - Real-time monitoring
Advanced anomaly detection tools continuously monitor network behavior, ensuring threats are identified in real time. This reduces the risk of prolonged security breaches. - Reducing false positives
Machine learning-based anomaly detection minimizes false alarms by improving accuracy over time, ensuring security teams focus on real threats. - Compliance and data protection
Many industries, such as finance and healthcare, are subject to strict data protection regulations. Anomaly detection helps organizations comply with security standards like GDPR and HIPAA.
Anomaly detection is the backbone of proactive cybersecurity. By identifying deviations early, organizations can prevent breaches that could cause catastrophic damage.
Mark Jenkins, a cybersecurity analyst
Also read: Exploring packet filters for anomaly detection in network security
Common applications of anomaly detection
1. Intrusion detection systems (IDS)
Anomaly detection forms the backbone of intrusion detection systems by identifying unauthorized access and flagging unusual traffic.
2. Fraud detection
Financial institutions use anomaly detection to identify suspicious transactions, such as credit card fraud or unauthorized withdrawals.
3. Malware and virus detection
By analyzing system behavior, anomaly detection tools can identify and isolate malware before it spreads.
4. Performance monitoring
Anomaly detection helps IT teams identify issues like latency, downtime, or hardware failures, ensuring smooth operations.

Technologies powering anomaly detection
- Artificial intelligence (AI)
AI algorithms analyze vast amounts of data, identifying anomalies with higher accuracy and speed. - Machine learning (ML)
Machine learning models continuously learn from network traffic to adapt to new behaviors, improving anomaly detection capabilities. - Big data analytics
Anomaly detection systems leverage big data analytics to process and analyze complex datasets, identifying trends and outliers. - Behavioral analytics
By understanding user behavior, systems can detect deviations that signal potential threats, such as unusual login times or data transfers.
Challenges in anomaly detection
- False positives
Anomaly detection systems may flag harmless deviations as threats, creating unnecessary alerts and overwhelming IT teams. - Resource requirements
Machine learning and AI-based systems require significant computational power and large datasets for training. - Evolving cyber threats
Sophisticated cybercriminals constantly develop techniques to bypass anomaly detection, requiring systems to adapt quickly. - Integration complexity
Implementing anomaly detection tools in existing IT infrastructure can be challenging, especially for legacy systems.
Also read: 5 difficulties in anomaly detection
Future trends in anomaly detection
- AI and deep learning
Advanced AI algorithms and deep learning models will improve anomaly detection accuracy, reducing false positives and detecting complex threats. - Cloud-based solutions
Cloud-based anomaly detection tools offer scalability and flexibility, enabling businesses to monitor networks efficiently. - Automated response systems
Integrating anomaly detection with automated response systems will allow networks to isolate threats immediately, minimizing damage. - Zero trust security
Anomaly detection will play a key role in zero trust security frameworks, ensuring every user and device is continuously monitored for suspicious activity.
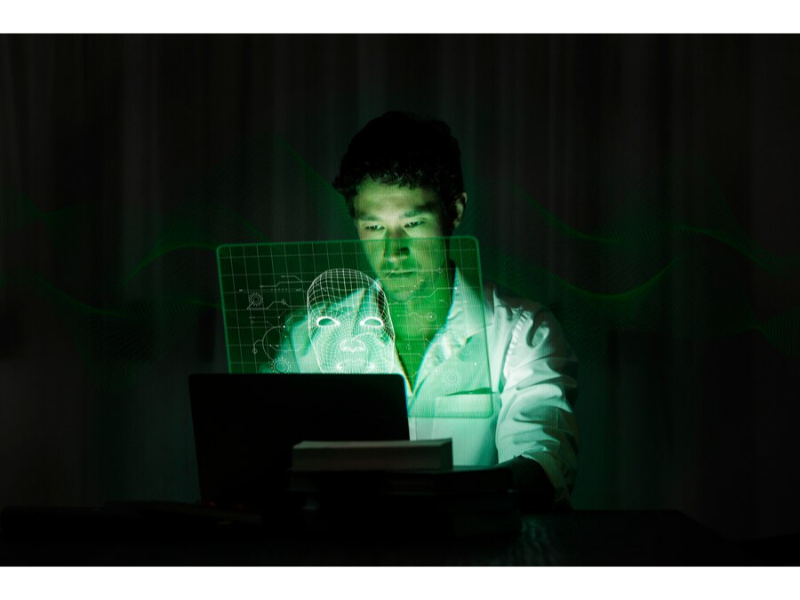
The integration of AI and behavioral analytics into anomaly detection tools is revolutionizing how we identify threats. The ability to process large volumes of data in real time gives organizations the edge they need to stay ahead of attackers.
David Collins, CTO of CyberNet Technologies
Anomaly detection is a cornerstone of modern network security, providing organizations with the tools to identify and mitigate threats in real time. By leveraging AI, machine learning, and big data analytics, anomaly detection systems are becoming more efficient and accurate.
As cyber threats continue to evolve, businesses must adopt robust anomaly detection strategies to protect their networks, data, and operations. From intrusion detection to fraud prevention, the applications of anomaly detection are vast, making it an essential component in any cybersecurity framework.
By addressing challenges such as false positives and resource demands, the future of anomaly detection lies in innovation and automation, ensuring networks remain secure and resilient.
Anomaly detection identifies unusual patterns in network traffic that deviate from normal behavior, helping detect potential security threats like malware or unauthorized access.
Machine learning algorithms analyze large datasets, learn from past behavior, and adapt to new patterns, improving accuracy in detecting anomalies.
Industries such as finance, healthcare, e-commerce, and telecommunications rely heavily on anomaly detection to protect sensitive data and ensure compliance with security regulations.
Challenges include false positives, resource requirements, adapting to evolving cyber threats, and integrating with existing IT systems.
Anomaly detection tools continuously analyze network activity, flagging deviations in real time to help security teams respond to threats quickly.