- Generative AI provides transformative solutions for drug discovery in the pharmaceutical and biotech industries.
- The McKinsey Global Institute estimates that this technology could generate $60 billion to US$110 billion in annual economic value for the pharmaceutical and medical products industry.
- By integrating generative AI, pharmaceutical companies can not only accelerate the discovery and development of new drugs but also enhance the customer experience and reduce the cost to market.
Artificial intelligence technology has enormous transformative potential in biotechnology and pharmaceuticals.
Generative AI has emerged as a transformative technology with the potential to revolutionise drug discovery. By harnessing the power of machine learning algorithms, generative AI in drug discovery promises to accelerate drug development, reduce costs, and ultimately save lives.
What is Generative AI?
Generative AI refers to a class of artificial intelligence techniques that are designed to generate new data samples that are similar to a given dataset.
Unlike traditional AI models that are trained to recognise patterns in data (such as classification or regression models), generative AI models are trained to understand and mimic the underlying distribution of the data.
When a user submits a query to a generative AI tool, the algorithm uses what it knows to construct a personalised response. It essentially predicts the high-level form of the text. The result is a human-like response rather than one that looks like it was generated by a robot.
While generative AI tools are often used to get simple answers, detailed and specific queries can generate longer, more thorough responses.
Also read: Artificial intelligence (AI) in everyday life
Where does generative AI fit into pharma companies?
Originating from relatively rudimentary applications in the 1950s, it has undergone a significant evolution. Owing to the exponential growth in data availability and computational power, its applications have dramatically diversified and complexified.
Generative AI is transforming almost every aspect of the pharmaceutical industry, changing the way companies operate and potentially unlocking billions of dollars in value.
The McKinsey Global Institute (MGI) estimates that the technology could generate between US$60 billion and US$110 billion in economic value for the pharmaceutical and medical products industry annually, largely because it could increase productivity in finding potential new drugs by accelerating the compound identification process, speeding up their development and approval, and improving how they are marketed.
Here’s how generative AI applies specifically in pharma companies.
Drug discovery: generative AI can help in the discovery phase by generating novel molecular structures with desired properties, such as efficacy and safety profiles.
These AI systems can explore vast chemical spaces much more efficiently than traditional methods, potentially leading to the discovery of new drug candidates.
Optimising drug design: once a potential drug candidate is identified, generative AI can assist in optimising its chemical structure to enhance its efficacy, specificity, and safety profile.
This process can involve designing analogues or derivatives with improved properties through iterative AI-driven simulations.
Predictive analytics: generative AI can analyse large datasets, including genomic data, clinical trial data, and real-world evidence, to identify patterns and predict drug responses in specific patient populations.
This can aid in personalised medicine approaches, allowing pharmaceutical companies to develop tailored treatments.
Formulation development: generative AI can assist in optimising drug formulations by predicting how different ingredients will interact and affect factors such as stability, bioavailability, and release kinetics.
This can streamline the formulation development process and lead to more effective drug delivery systems.
Generative chemistry: AI-driven generative chemistry platforms can rapidly generate chemical compounds with desired properties, enabling high-throughput screening and accelerating the identification of lead compounds for drug development projects.
Patent analysis and intellectual property: generative AI can analyse patent databases and scientific literature to identify gaps in the intellectual property landscape, helping pharmaceutical companies make informed decisions about patenting strategies and avoid potential infringement issues.
Drug repurposing: generative AI can analyse existing drugs and their molecular properties to identify new therapeutic uses or potential combinations for repurposing existing drugs.
This approach can significantly shorten the drug development timeline and reduce costs compared to traditional de novo drug discovery.
Adverse event prediction: by analysing real-world data and adverse event reports, generative AI can help pharmaceutical companies identify potential safety issues with drugs early in the development process, enabling proactive risk mitigation strategies.
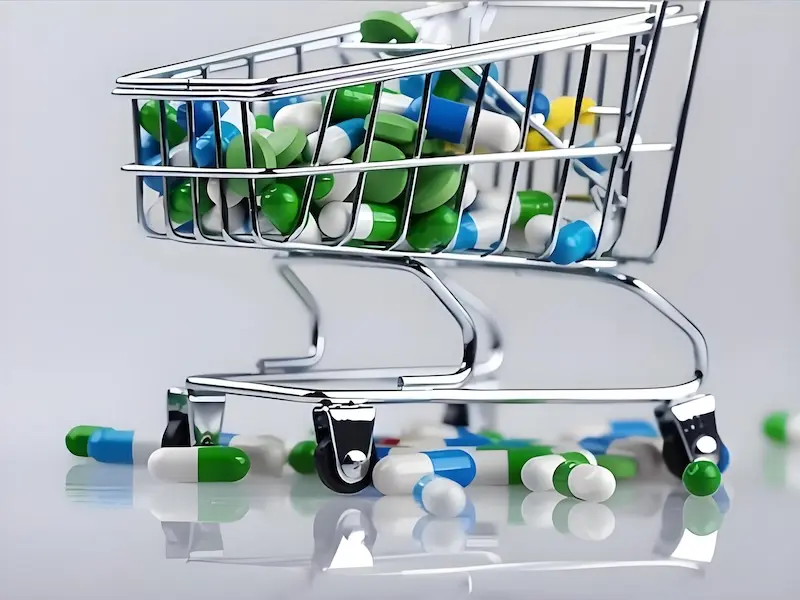
Generative AI use cases in pharmaceuticals and biotechnology
Generative AI holds promise across various domains within pharmaceuticals and biotechnology.
Here are some specific use cases.
Molecule generation: generative AI can be used to generate novel molecular structures with desired properties, such as binding affinity to a target protein or specificity for a particular biological pathway.
This can accelerate the discovery of new drug candidates by exploring vast chemical spaces more efficiently than traditional methods.
Lead optimisation: once a lead compound is identified, generative AI can assist in optimising its chemical structure to improve its potency, selectivity, and pharmacokinetic properties.
This iterative process can lead to the development of more effective and safer drug candidates.
Drug repurposing: generative AI can analyse the molecular structures of existing drugs and predict their potential efficacy against different diseases or targets.
This approach can identify new therapeutic uses for approved drugs, accelerating the development of treatments for unmet medical needs.
Biomolecule engineering: generative AI can design novel peptides, proteins, antibodies, or enzymes with desired properties, such as enhanced binding affinity, stability, or specificity.
This can facilitate the development of biologics for various therapeutic and diagnostic applications.
Compound synthesis planning: generative AI can propose synthetic routes for the production of target molecules, taking into account factors such as chemical feasibility, cost, and environmental impact.
This can streamline the synthesis process and enable the efficient production of complex compounds.
Biomedical imaging analysis: generative AI can analyse medical imaging data, such as MRI or CT scans, to identify biomarkers, predict disease progression, or assist in treatment planning.
This can improve diagnosis accuracy and patient outcomes in areas such as oncology, neurology, and cardiology.
Genomics and drug response prediction: Generative AI can analyse genomic data to identify genetic markers associated with drug response or adverse reactions.
This information can inform personalised medicine approaches, enabling the selection of treatments tailored to individual patients based on their genetic profile.
Clinical trial optimisation: generative AI can analyse clinical trial data to optimise trial design, patient recruitment, and endpoint selection.
By identifying factors that influence trial outcomes, researchers can improve the efficiency and success rate of clinical studies.
Drug safety and toxicity prediction: generative AI can predict the potential safety and toxicity profiles of drug candidates based on their chemical structure and biological properties.
This can help prioritise lead compounds with favourable safety profiles and mitigate the risk of adverse effects during development.
Also read: OpenAI and Meta to launch AI models with ‘reasoning’ capabilities
Challenges of using generative AI in pharma
Despite the growing role of generative AI in the pharmaceutical industry, there are several challenges to using generative AI in the biotech and pharmaceutical industries. For example, generative AI does not work out of the box.
Here comes several challenges.
Data quality and availability: generative AI models require large, diverse, and high-quality datasets to learn effectively.
However, in the pharmaceutical domain, such datasets may be limited, especially for rare diseases or novel drug targets. Ensuring data quality, standardisation, and accessibility remains a challenge.
Bias and generalization: generative AI models trained on biased or incomplete datasets may produce biased or unrealistic outputs.
Ensuring fairness, diversity, and generalization capabilities of generative models is crucial to avoid unintended consequences, especially in personalized medicine and patient-specific treatment approaches.
Validation and verification: validating the accuracy, reliability, and safety of generative AI-generated results is challenging, particularly in the context of drug discovery and development.
Rigorous experimental validation and verification processes are necessary to ensure that generated molecules or predictions meet the required standards for efficacy and safety.