- The exponential growth of AI, exemplified by ChatGPT, is projected to lead to a significant surge in energy demand, potentially consuming up to 25% of U.S. electricity by 2030, sparking concerns of a looming energy crisis and triggering a global “energy war.”
- To address the escalating energy consumption of AI, strategies such as optimising large models and AI hardware to reduce energy consumption, as well as investing in new energy sources like nuclear fusion, are being pursued by industry experts and major tech players like Amazon, Google, and Altman.
- Despite the challenges posed by AI’s energy consumption, optimism remains regarding the capacity to meet demand, with ongoing advancements in renewable energy, infrastructure upgrades, and international collaboration aimed at ensuring a sustainable energy future amidst the AI revolution.
As ChatGPT triggers a new wave of artificial intelligence (AI) fever, the underlying issue of energy consumption continues to attract attention.
On April 10 this year, Rene Haas, CEO of chip giant Arm, publicly stated that large AI models like ChatGPT require significant computational power. It is estimated that by 2030, AI data centres will consume 20% to 25% of the electricity demand in the United States, a significant increase from today’s 4%.
Public data shows that ChatGPT currently processes over 200 million requests per day, consuming up to 500,000 kilowatt-hours of electricity daily. This translates to an annual electricity bill of 200 million RMB for ChatGPT alone.
This means that the daily electricity consumption of ChatGPT is over 17,000 times that of an average household. (Commercial electricity in the United States is currently around $0.147 per kilowatt-hour, equivalent to 1.06 yuan, or 530,000 yuan per day)
According to Alex de Vries, head of a Dutch consulting firm, the AI industry is expected to consume 850 billion to 1,340 billion kilowatt-hours of electricity per year by 2027, equivalent to the annual total electricity consumption of a European country like Sweden or the Netherlands.
Musk predicts that the power shortfall may occur as early as 2025, stating, “Next year you’ll see, we won’t have enough power to run all the chips.”
OpenAI CEO Sam Altman also anticipates an energy crisis in the AI industry, suggesting that future AI technological development will heavily rely on energy, and people will need more photovoltaic and energy storage products.
All of this indicates that AI is on the verge of igniting a new global “energy war”.
Also read: 5 women that are changing the AI industry
Also read: What AI voice generator is everyone using?
AI hits an energy bottleneck
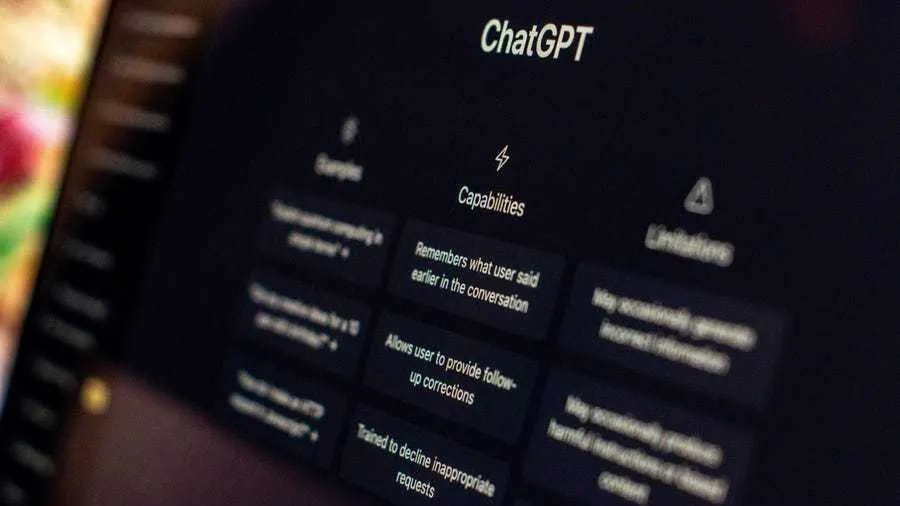
Over the past 500 days, ChatGPT has sparked a global surge in demand for large AI models and computing power.
Major global tech giants like Microsoft, Google, Meta, and OpenAI have been scrambling to acquire AI chips, even venturing into chip manufacturing themselves, with a total scale exceeding tens of trillions of dollars.
In essence, AI relies heavily on computer technology and information processing, which in turn requires a significant number of GPU chips, as well as underlying resources such as electricity, hydropower, wind energy, and funding.
As early as 1961, physicist Rolf Landauer, working at IBM, published a paper proposing what later became known as “Landauer’s Principle”.
This theory suggests that when information stored in a computer undergoes irreversible changes, it emits a small amount of heat to the surrounding environment, with the amount of heat emitted depending on the temperature of the computer at the time – the higher the temperature, the more heat is emitted.
Landauer’s Principle links information and energy, specifically to the second law of thermodynamics. Because logically irreversible information processing operations result in the annihilation of information, this leads to an increase in entropy in the physical world, thus consuming energy.
Since its proposal, this principle has faced considerable skepticism. However, in the past decade or so, “Landauer’s Principle” has been experimentally verified.
In 2012, a study published in Nature measured for the first time the minute amount of heat released when a “bit” of data is deleted. Subsequent independent experiments have also confirmed “Landauer’s Principle”.
Over the past decade, modern electronic computers have consumed energy in computation billions of times higher than the theoretical value of Landauer’s principle. Scientists have been striving to find more efficient computing methods to reduce costs.
Today, with the explosion of large AI models, there is indeed a significant need for computation. Therefore, AI is not only constrained by chip shortages but also by energy shortages.
Recently, at the ‘Bosch Connected World 2024‘ conference, Musk also stated that over a year ago, the shortage was in chips, but next year you will see a shortage of electricity, unable to meet the demand for all chips.
Li Xiuquan, Deputy Director of the Artificial Intelligence Centre at the Chinese Academy of Science and Technology Information Institute, also stated, “in recent years, the scale and quantity of large AI models have been growing rapidly, leading to a rapid increase in energy demand. While issues like “power shortages” are unlikely to arise quickly in the short term, the exponential increase in energy demand with the advent of the era of large-scale intelligence cannot be ignored.”
The key to the quality of AI large models lies in data, computing power, and top talent, supported by the continuous operation of tens of thousands of chips day and night.
Specifically, the working process of AI model computing power can be roughly divided into two stages: training and inference, and energy consumption is also involved.
A large amount of text data needs to be collected and preprocessed during training
In recent years, the scale and quantity of large AI models have been growing rapidly, leading to a rapid increase in energy demand. While issues like ‘power shortages’ are unlikely to arise quickly in the short term, the exponential increase in energy demand with the advent of the era of large-scale intelligence cannot be ignored.
Li Xiuquan, Deputy Director of the Artificial Intelligence Centre at the Chinese Academy of Science and Technology Information Institute
During the training phase, a large amount of text data needs to be collected and preprocessed as input data. Next, the model parameters are initialised in an appropriate model architecture, the input data is processed, and attempts are made to generate output. Finally, the parameters are repeatedly adjusted based on the difference between the output and the expected output until the performance of the model no longer significantly improves.
From training GPT-2 with 1.5 billion parameters to training GPT-3 with 175 billion parameters, the training energy consumption behind OpenAI models is astonishing. Public information indicates that OpenAI consumes 128.7 kWh per training, equivalent to 32 km traveled by 3000 Teslas simultaneously.
According to research firm New Street Research, Google alone requires about 400,000 servers for AI, consuming 62.4 GWh per day and 22.8 TWh per year.
During the inference phase, AI first loads the trained model parameters, preprocesses the text data that needs to be inferred, and then allows the model to generate output based on the learned language patterns. Google claims that from 2019 to 2021, 60% of AI-related energy consumption comes from inference.
According to Alex de Vries’ estimation, ChatGPT consumes over 500,000 kWh per day to respond to approximately 200 million requests, resulting in an annual electricity bill of 200 million RMB, which is 1.7 times higher than the average daily electricity consumption of each American household.
A report from SemiAnalysis states that the energy consumption for problem searching using large models is ten times that of conventional keyword searches. Taking Google as an example, standard Google searches use 0.3Wh of power, while each interaction with large models consumes 3Wh. If users use AI tools for every Google search, it would require approximately 29.2 TWh of electricity per year, or about 79 million kWh per day. This is equivalent to providing continuous power to the world’s largest skyscraper, the Burj Khalifa in Dubai, for over 300 years.
Furthermore, according to the Stanford Artificial Intelligence Index Report 2023, each AI search consumes approximately 8.9 Wh of electricity. Compared to regular Google searches, the energy consumption per AI search is nearly 30 times higher. For a model with up to 176 billion parameters, the training phase alone consumes 433,000 kWh, equivalent to the annual electricity consumption of 117 American households.
It is worth noting that within the Scaling Law, as the scale of parameters continues to increase, the performance of large models also improves, accompanied by higher energy consumption.
Therefore, the energy issue has become a critical “shackle” for the continuous development of AI technology.
Pop quiz
How many servers does Google require for AI?
A. 400,000
B. 300,000
C. 200,000
D. 100,000
The correct answer is at the bottom of the article.
It is still far from catastrophic
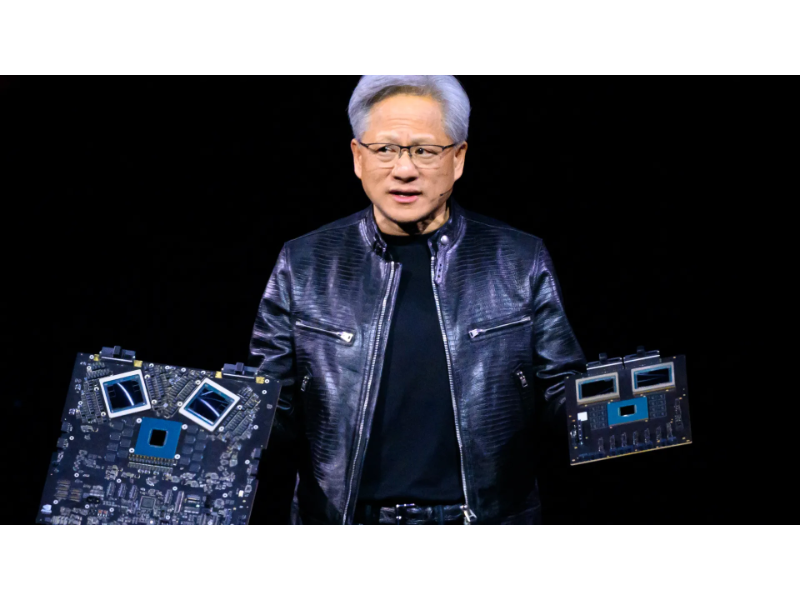
Jensen Huang, despite his concerns about energy supply, offers a more optimistic view: over the past decade, AI computing has increased by a millionfold, yet its costs, space, or energy consumption have not grown by the same magnitude.
According to the U.S. Energy Information Administration’s (EIA) long-term annual outlook, the annual growth rate of U.S. electricity demand is less than 1% currently. However, John Ketchum, CEO of NextEra Energy, estimates that under the influence of AI technology, this annual growth rate of electricity demand will accelerate to around 1.8%.
A report from the Boston Consulting Group shows that in 2022, data centre electricity consumption accounted for 2.5% of total U.S. electricity consumption (about 130 terawatt-hours), and is expected to double by 2030 to reach 7.5% (about 390 terawatt-hours). This is equivalent to the electricity consumption of about 40 million American households, or one-third of all American households. The group also predicts that generative AI will account for at least 1% of the new electricity demand in the United States.
This means that even though the electricity consumption of data centres and AI is significant, it is far from catastrophic.
In terms of cost, a report from the International Renewable Energy Agency pointed out that over the past decade, the average cost of electricity for global wind and solar power projects has cumulatively decreased by over 60% and 80%, respectively. Industry insiders also mentioned, “The comprehensive cost of photovoltaics is similar to that of thermal power, while wind power costs half as much in some regions as thermal power.”
How will we cope with the upcoming surge in energy demand?
According to summaries from industry experts by the Titanium Media App, there are two main solutions to addressing AI energy consumption: one is to reduce energy consumption through optimisation of large models or AI hardware, and the other is to find new energy sources, such as nuclear fusion, fission resources, etc., to meet the energy demands of AI.
In terms of hardware optimisation, for trillion-level AI large models with high energy consumption, energy consumption can be reduced by compressing model token size and complexity through algorithm and model optimisation. At the same time, enterprises can continue to develop and update AI hardware with lower energy consumption, such as the latest NVIDIA B200, AI PCs, or AI phone terminals. In addition, by optimising the energy efficiency of data centres and improving the efficiency of power usage, energy consumption can be reduced.
In response to this, Bai Wenxi, Vice Chairman of the China Enterprise Capital Alliance, stated, “in the future, there is a need for technological innovation and equipment upgrades to further improve power generation efficiency, increase grid transmission capacity and stability, optimise power resource allocation, enhance the flexibility of power supply, promote distributed energy systems, and reduce energy transmission losses to cope with the energy demand challenges brought about by computing power development.”
Qu Haifeng, Deputy Director of the China Data Centre Working Group (CDCC) Expert Committee, believes that relevant industries should focus on improving the energy efficiency of data centres rather than suppressing their scale. Data centres do not need to reduce energy consumption, but rather improve the quality of energy consumption.
As for the development of nuclear fusion energy, due to its abundant raw material resources, large energy release, safety, cleanliness, and environmental advantages, controlled nuclear fusion can basically meet various requirements for the ideal ultimate energy source in the future.
There are currently three main sources of fusion energy: cosmic energy, such as solar light and heat; hydrogen bomb explosion (uncontrolled nuclear fusion); artificial sun (controlled nuclear fusion energy device).
According to statistics, there are currently more than 50 countries in the world conducting research and construction of over 140 fusion devices, and a series of technological breakthroughs have been made. The IAEA expects the world’s first fusion power plant to be built and put into operation by 2050.
This fusion power generation will greatly alleviate the global energy shortage caused by the demand for AI large models.
In April 2023, Altman took preemptive action by personally investing $375 million in the fusion startup company Helion Energy and served as the company’s chairman. Additionally, Altman merged AltC, his investment company, with the fusion startup company Oklo last July, securing an IPO valued at approximately $850 million, with AltC’s latest market capitalisation exceeding $40 billion.
In addition to Altman’s heavy investment in fusion companies, tech giants like Amazon and Google are directly purchasing clean energy.
According to Bloomberg data, in 2023 alone, Amazon purchased 8.8 gigawatts (GW) of clean energy, marking the fourth consecutive year it has become the world’s largest corporate buyer of clean energy. Meta (3 GW) and Google (1 GW) follow closely behind.
Amazon states that over 90% of its data centre electricity comes from clean energy generation and is expected to achieve 100% green energy usage by 2025.
The competition between China and the United States
In fact, taking the United States as an example, the growth of multiple industries such as clean energy, AI, data centres, electric vehicles, and mining has revived the stagnant U.S. electricity demand. However, even hailed as the ‘largest machine’ in the world, the U.S. power grid seems unable to cope with this sudden change.
Analysts point out that 70% of the U.S. grid access and transmission facilities are aging, and some areas have insufficient grid transmission lines. Therefore, the U.S. power grid needs massive upgrades, and if no action is taken, the United States will face an insurmountable domestic supply gap by 2030.
Compared to the United States, China is more optimistic about its energy demand. Currently, China’s wind power and photovoltaic products have been exported to more than 200 countries and regions, with cumulative exports exceeding $33.4 billion and $245.3 billion, respectively.
As AI experiences explosive growth, the competition between China and the United States in the AI industry has evolved from a competition in large model technology to a multi-faceted battle involving computing power, energy, manpower, and more.
With the potential deployment of nuclear fusion energy by 2050, humanity hopes to end the challenging problem of AI energy consumption and enter an era of unlimited power generation.
The correct answer is A.