- Big data analytics is the process of examining vast and diverse datasets to uncover patterns, correlations, and insights that inform decision-making.
- Big data analytics features include large-scale volume, high velocity, diverse variety, real-time processing, advanced machine learning, scalability, data integration, and actionable insights.
What is big data
Big data refers to extremely large and complex sets of data that are difficult to process and analyze using traditional data-processing software. Watch this video below to understand types of big data.
Big data analytics
Big data analytics is a field that leverages advanced tools and techniques to process and analyze vast, complex datasets, uncovering patterns, trends, and insights that inform decision-making. In a world increasingly driven by data, the volume, velocity, and variety of information produced every second continue to grow.
From social media interactions and transaction records to sensor data from IoT devices, organizations generate massive amounts of data in real time. Traditional data processing methods are often insufficient to handle this scale and complexity, making big data analytics essential for transforming raw data into valuable insights.
Also read: Encryption and data size: What’s the impact?
Big data features
1. Volume
Big data refers to extremely large datasets—ranging from terabytes to zettabytes—that require advanced tools to store, manage, and analyze.
2. Velocity
Big data analytics handles data that’s generated and processed at high speeds, often in real time. Examples include social media updates, IoT sensor data, and financial market data.
3. Variety
Big data includes diverse data types such as structured (e.g., databases), semi-structured (e.g., XML files), and unstructured data (e.g., text, video, audio), which all require specialized techniques for processing.
Also read:Data lakes 101: What is a data lake and how does it work?
4. Veracity
Big data analytics also tackles issues of data quality, accuracy, and reliability. It involves cleaning and validating data to ensure the insights derived are trustworthy.
5. Value
The ultimate goal of big data analytics is to extract actionable insights that can lead to business improvements, customer satisfaction, cost savings, or other value-added benefits.
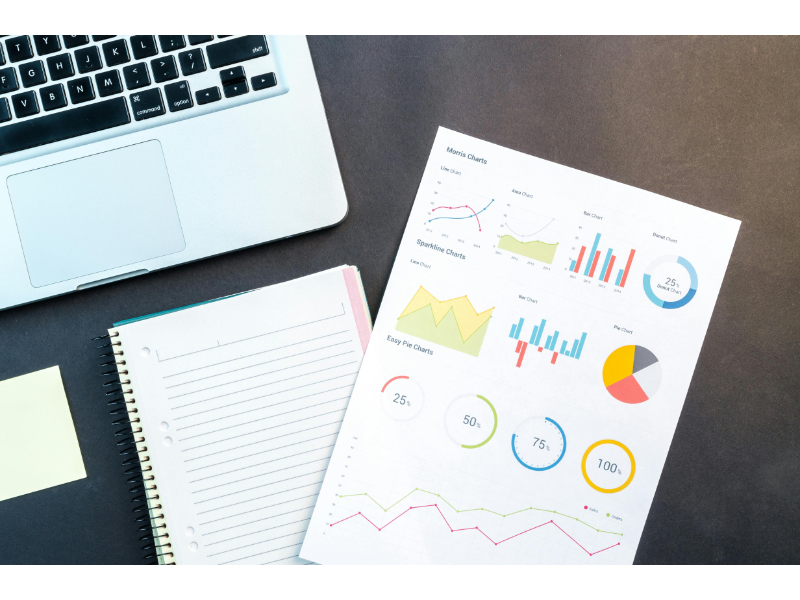
6. Advanced Analytics Techniques
It involves techniques like machine learning, predictive modeling, data mining, and artificial intelligence to uncover hidden patterns, correlations, and trends.
7. Scalability
Big data analytics systems must be highly scalable, as they need to expand with data volume, variety, and velocity. Cloud computing and distributed processing frameworks (e.g., Hadoop, Spark) enable this scalability.
8. Real-time analytics
Many big data analytics platforms support real-time processing, allowing for immediate insights and timely decision-making in sectors like finance, healthcare, and e-commerce.
9. Data integration
Big data analytics involves integrating data from multiple sources to form a cohesive and comprehensive view. This is crucial for producing insights that are holistic and contextually relevant.
10. Automation
Many big data solutions include automation features that streamline processes such as data collection, analysis, and reporting. Automation enhances efficiency and reduces the time required to generate insights from large datasets.
These features make big data analytics a powerful approach for handling and deriving insights from massive and complex datasets.