- Narrow AI refers to artificial intelligence systems designed to perform specific tasks with high efficiency, such as voice assistants and facial recognition.
- Unlike Artificial General Intelligence (AGI), Narrow AI is limited to its predefined functions and lacks the ability to adapt or handle tasks outside its specialty.
Artificial Intelligence (AI) has evolved significantly over the last few decades, and while many people associate it with futuristic, human-like robots, the reality of AI today is far more specialized. The term “Narrow AI,” also known as Weak AI, refers to AI systems designed to perform a specific task or a narrow range of tasks with high efficiency. Unlike General AI, which aspires to mimic human cognitive functions across various domains, Narrow AI is focused on solving specific problems.
In this article, we will dive deep into the concept of Narrow AI, its applications, benefits, and challenges, as well as its place in the broader landscape of artificial intelligence technologies.
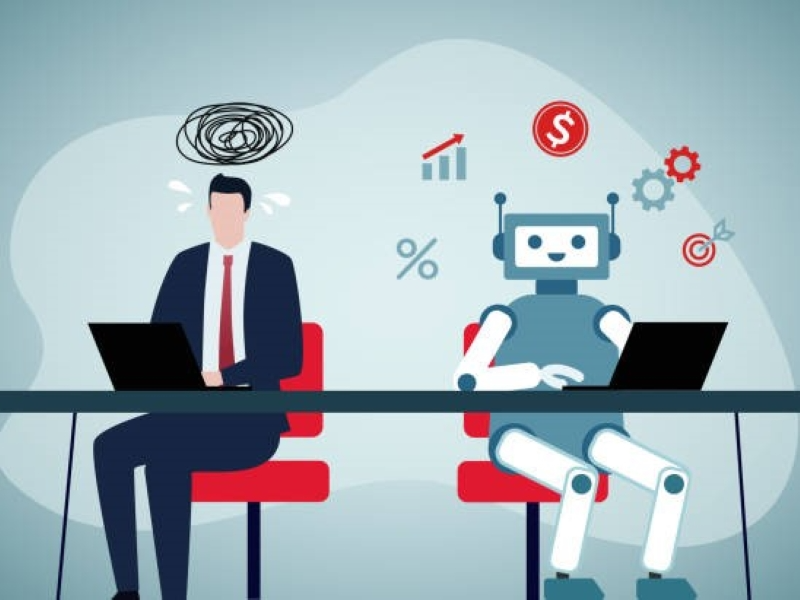
Also read: Understanding narrow AI: Specialised intelligence in focus
Also read: 4 advantages of narrow AI: Unlocking efficiency and precision
What is narrow AI?
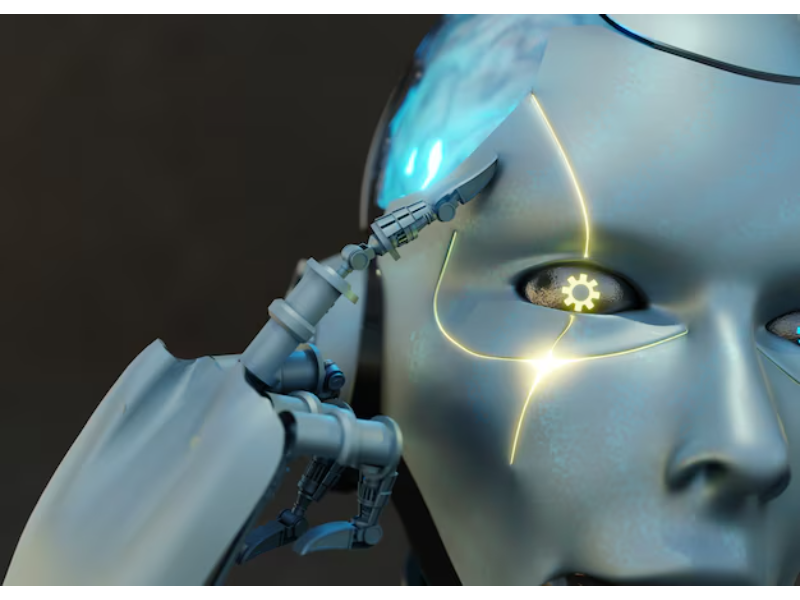
Narrow AI refers to artificial intelligence that is programmed to perform a specific task or a limited range of tasks. It’s often designed with a narrow focus and excels in that area but lacks the ability to handle tasks outside its programmed domain. Essentially, Narrow AI performs tasks such as image recognition, speech processing, or playing chess, but it doesn’t possess general intelligence or self-awareness.
The term Weak AI is commonly used in contrast to Strong AI or Artificial General Intelligence (AGI). While Strong AI envisions systems that can reason, plan, and learn autonomously in multiple domains, Narrow AI is confined to predefined functions. Most of the AI technologies used in our daily lives today fall under the Narrow AI category. According to Stuart Russell, a leading AI researcher and author of Artificial Intelligence: A Modern Approach, “Narrow AI has achieved remarkable progress in recent years, but it remains fundamentally constrained by its design, focusing on specific tasks rather than general problem-solving.”
Geoffrey Hinton, a pioneer in machine learning, further clarifies, “Narrow AI is incredibly effective within its defined scope, but it cannot adapt or apply its knowledge across different domains without retraining or reprogramming.” In addition, Yoshua Bengio, another deep learning expert, explains, “While Narrow AI has brought great benefits to sectors like healthcare and autonomous driving, it is far from achieving the versatility of human-like intelligence, which is the goal of AGI.”
Narrow AI has achieved remarkable progress in recent years, but it remains fundamentally constrained by its design, focusing on specific tasks rather than general problem-solving.
Staurt Russell, a leading AI researcher and author of Artificial Intelligence: A Modern Approach
Also read: 10 examples of narrow AI
Also read: Narrow AI vs. general AI: What’s the real difference?
The difference between narrow AI and artificial general intelligence (AGI)
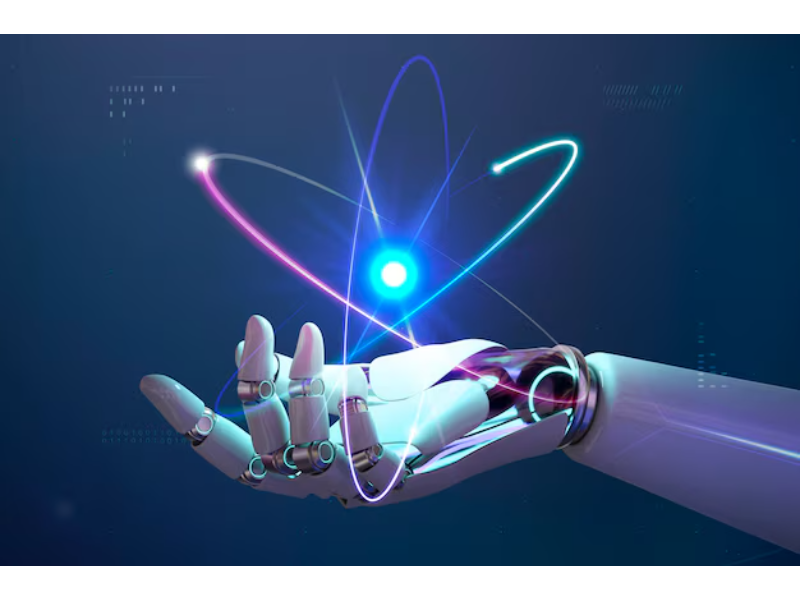
One of the most common misconceptions about AI is confusing Narrow AI with Artificial General Intelligence (AGI). AGI refers to an AI system capable of performing any intellectual task that a human being can do. It can generalize its learning to different areas, think abstractly, and adapt to new situations. This is still a distant goal for AI researchers and hasn’t yet been realized. Demis Hassabis, CEO of DeepMind, adds: “Narrow AI systems are incredibly good at specialized tasks, but their lack of general understanding or ability to transfer knowledge makes them fundamentally different from the broader intelligence we see in humans.”
On the other hand, Narrow AI is task-specific. It doesn’t possess any cognitive abilities beyond its programmed scope. For example, Siri or Alexa can assist with voice commands but can’t engage in conversations outside their predefined functionalities. Narrow AI doesn’t “think” the way humans do—it simply processes data and executes algorithms designed for particular purposes. As Fei-Fei Li, a leading AI researcher at Stanford University, explains: “Narrow AI is a powerful tool in solving specific problems, but it lacks the ability to reason or adapt beyond its defined limits.”
Narrow AI systems are incredibly good at specialized tasks, but their lack of general understanding or ability to transfer knowledge makes them fundamentally different from the broader intelligence we see in humans.
Demis Hassabis, CEO of DeepMind
Also read: Understanding narrow AI: Is ChatGPT a good example?
Also read: What is general AI? Understanding the next evolution in AI
Key characteristics of narrow AI
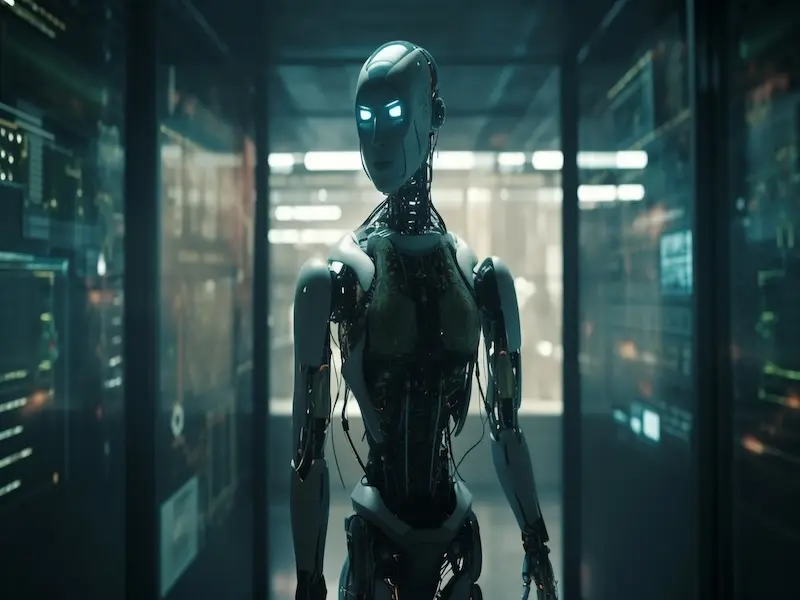
Narrow AI systems are characterized by several key features:
- Specialization: Narrow AI is built to excel at a specific task. It can outperform humans in these areas by processing vast amounts of data much faster and more accurately.
- Task-Specific: It is programmed to do one thing very well, such as facial recognition, recommendation algorithms, or autonomous vehicles. The system cannot apply its learned knowledge to tasks outside its specialty.
- Data-Driven: Narrow AI relies heavily on data to function. Machine learning, a subset of AI, allows it to improve over time as it is exposed to more data.
- No Autonomy: It doesn’t have the capacity for independent decision-making or reasoning across different domains. It cannot think or innovate outside its specific field of expertise.
Also read: Is AI ready to be a general purpose technology?
Examples of narrow AI
The real-world applications of Narrow AI are widespread, and many of us interact with it daily without realizing it. Some of the most common examples of Narrow AI include:
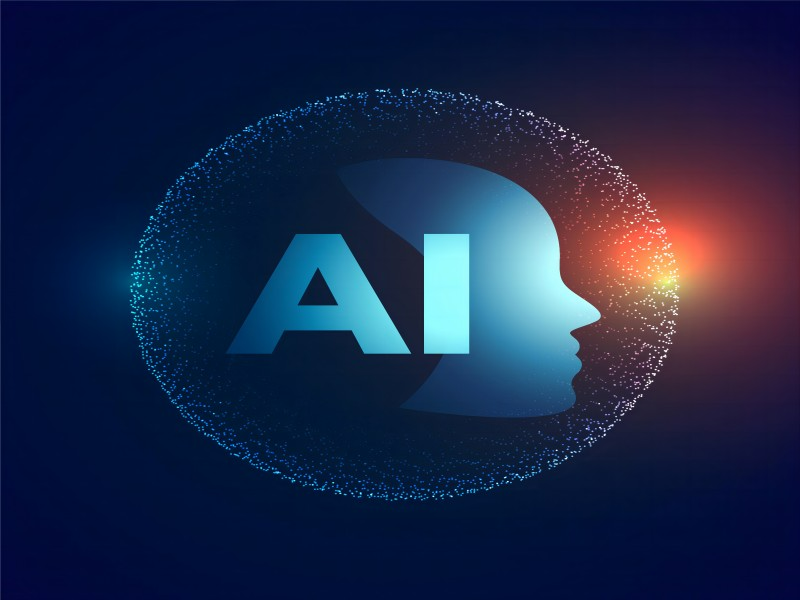
1. Voice assistants
Voice assistants like Amazon Alexa, Apple Siri, and Google Assistant are prime examples of Narrow AI. These systems can perform tasks such as setting alarms, answering questions, and controlling smart home devices. However, their capabilities are limited to these tasks and don’t extend beyond the range of commands they are programmed to handle.
2. Recommendation systems
Recommendation algorithms used by platforms such as Netflix, Spotify, and Amazon are powered by Narrow AI. These systems analyze your preferences and behaviors to suggest movies, music, or products that align with your tastes. While these systems are highly specialized, they can’t recommend products or services outside the scope of their programming.
3. Autonomous vehicles
Self-driving cars, like those developed by Tesla and Waymo, use Narrow AI for functions like navigation, obstacle detection, and decision-making. These systems are designed to perform specific tasks related to driving, such as detecting pedestrians, following traffic rules, and adjusting speed.
4. Chatbots
AI-driven chatbots used for customer service, such as Zendesk, Drift, and Intercom, are examples of Narrow AI. These chatbots can handle customer queries, provide troubleshooting advice, and even process transactions. However, they are limited to predefined scenarios and cannot handle more complex or unscripted conversations.
5. Facial recognition
Facial recognition technology, used for security purposes and in applications such as Apple Face ID, leverages Narrow AI to identify individuals based on their facial features. These systems are highly accurate at matching faces within their specific domain but cannot generalize to other tasks, such as voice recognition or object detection.
Benefits of narrow AI
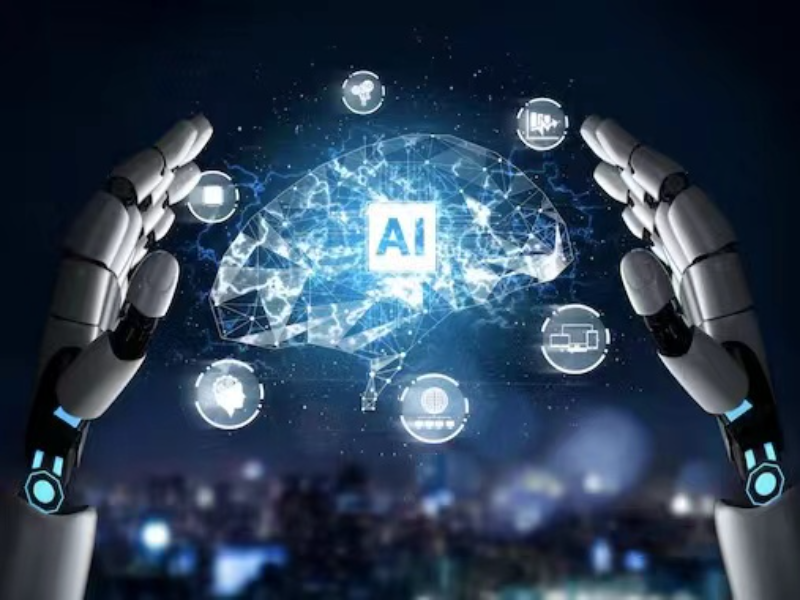
Narrow AI provides several key advantages, particularly in terms of efficiency, scalability, and problem-solving capabilities. Some of the notable benefits include:
1. Efficiency
Narrow AI systems can process massive amounts of data far more quickly than humans. For example, AI-powered medical imaging systems can analyze X-rays or MRIs at a much faster rate than human doctors, potentially identifying abnormalities that might be overlooked in manual examinations.
2. Accuracy
AI systems, when trained with quality data, can achieve a high level of accuracy in their specific tasks. In areas like fraud detection or financial analysis, Narrow AI can quickly identify patterns that human analysts might miss, minimizing errors and increasing overall precision.
3. Cost-Effectiveness
Since Narrow AI is typically focused on a single task, it can reduce the need for human labor in repetitive or mundane jobs. This can lead to significant cost savings for companies in industries like customer service, manufacturing, and retail.
4. Scalability
Narrow AI systems can be easily scaled to handle growing amounts of data or increased demand. For instance, as e-commerce platforms expand, their recommendation engines can process larger datasets to suggest more personalized products to a broader audience.
5. Automation
Narrow AI plays a key role in automating repetitive and time-consuming tasks. This is evident in industries like manufacturing, where AI-powered robots perform tasks such as assembling products, or in logistics, where AI algorithms optimize delivery routes.
Challenges of narrow AI
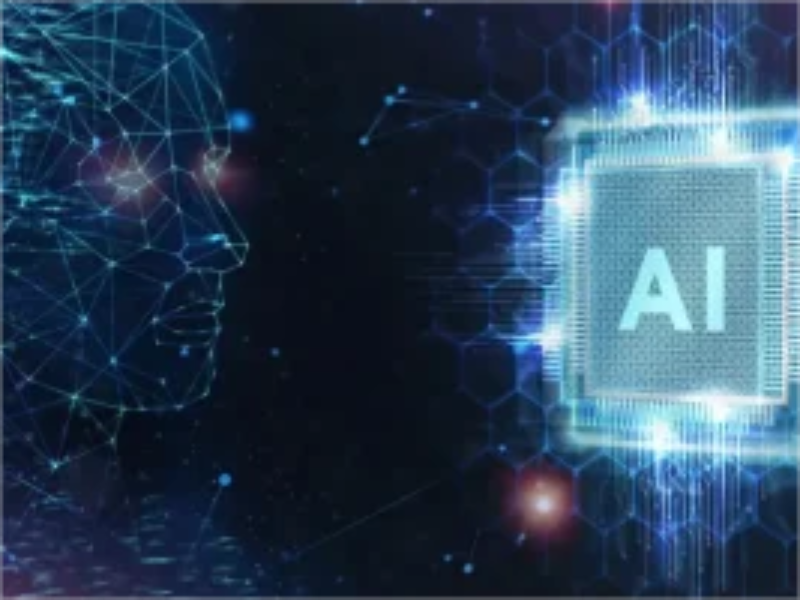
While Narrow AI offers numerous advantages, it also comes with its own set of challenges. Some of these challenges include:
1. Limited adaptability
Narrow AI is only effective within its specific domain. It cannot adapt to new tasks or solve problems outside its training scope. For example, a facial recognition system trained to identify faces cannot be repurposed for recognizing objects or understanding natural language. As Yoshua Bengio, a deep learning pioneer, notes: “Narrow AI’s inability to transfer knowledge from one task to another highlights its limits—its functionality is strictly confined to the scope it was trained for, unlike human intelligence, which is adaptable.”
2. Data dependency
Narrow AI systems require large amounts of high-quality data to function effectively. In many cases, if the data is biased or incomplete, the AI system can make inaccurate predictions or decisions. This issue is particularly concerning in fields like criminal justice, where biased AI systems may perpetuate existing prejudices. Kate Crawford, a leading researcher in AI and ethics, emphasizes, “The power of data is central to Narrow AI’s effectiveness, but we must recognize that biased data can lead to harmful outcomes, especially when AI is used for critical decisions, such as in law enforcement or hiring.”
3. Ethical concerns
The deployment of Narrow AI systems, particularly in areas like facial recognition, raises significant ethical concerns. Privacy issues, bias in decision-making, and the potential for misuse are all important considerations when implementing AI technologies. Timnit Gebru, an AI ethics researcher, argues, “Narrow AI, especially when used for surveillance, has the potential to infringe on privacy rights, and it’s critical that we address these ethical concerns before deploying these systems at scale.”
4. Job displacement
As Narrow AI continues to automate tasks, there are concerns about job displacement, especially in industries like manufacturing, customer service, and retail. While AI can improve productivity, it can also reduce the need for human workers in certain roles. Kai-Fu Lee, a leading AI expert and author of AI Superpowers, warns: “While Narrow AI can increase productivity, it also has the potential to displace millions of jobs, especially in routine and manual labor sectors. We must prepare for a future where workers need retraining and reskilling to adapt to AI-driven economies.”
Narrow AI’s inability to transfer knowledge from one task to another highlights its limits—its functionality is strictly confined to the scope it was trained for, unlike human intelligence, which is adaptable.
Yoshua Bengio, a deep learning pioneer
Also read: How will AI ethics impact society?
Narrow AI in the future
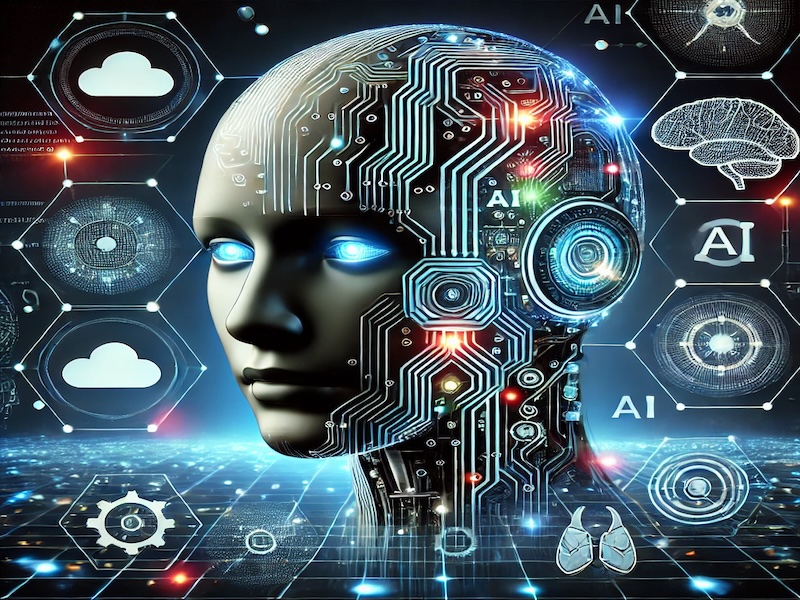
While Narrow AI is currently the most prevalent form of AI, it will continue to evolve and may eventually play a significant role in advancing toward Artificial General Intelligence. Researchers are exploring ways to make AI systems more flexible, capable of learning from smaller datasets, and able to generalize across different tasks.
In the coming years, we are likely to see hybrid AI systems that combine the strengths of Narrow AI with broader capabilities. These systems may be able to handle more complex tasks while still retaining the efficiency and accuracy that Narrow AI is known for.
FAQ
Narrow AI, also known as Weak AI, refers to AI systems that are designed to perform a specific task or a limited range of tasks, such as facial recognition or voice assistants. Unlike Artificial General Intelligence (AGI), which aims to simulate human-like intelligence across a wide range of domains, Narrow AI operates within a specialized area and cannot adapt to new tasks without specific programming or retraining.
Yes, Narrow AI can improve through techniques such as machine learning and deep learning. By processing more data, these systems can become more accurate and efficient at their designated tasks. However, its improvement is still limited to the scope it was designed for and cannot be transferred to unrelated tasks without retraining.
Some common examples of Narrow AI include:
Voice assistants like Amazon’s Alexa or Apple’s Siri, which handle specific tasks like setting alarms or playing music.
Recommendation systems on platforms like Netflix or Spotify, which suggest content based on your preferences.
Facial recognition technology used in security systems and smartphone unlocking.
Autonomous vehicles, where AI systems handle driving tasks like navigation and obstacle detection.
While Narrow AI is highly effective at its specific tasks, it has several limitations:
Limited adaptability: It cannot generalize its learning to other tasks or domains.
Data dependency: It relies on large volumes of high-quality data to function correctly. Poor or biased data can lead to inaccurate results.
Lack of cognitive flexibility: Unlike human intelligence, Narrow AI lacks the ability to reason, abstract, or think creatively outside its predefined functions.
Narrow AI is revolutionizing industries by automating repetitive tasks, improving efficiency, and enabling new capabilities. However, it also poses challenges, such as potential job displacement in sectors like customer service, retail, and manufacturing. While it can reduce labor costs, it may require workers to undergo retraining or reskilling to remain competitive in the job market.