- AI algorithms, including machine learning and natural language processing, enhance threat detection by analysing data for patterns and anomalies.
- Anomaly detection in cybersecurity leverages AI systems to detect abnormal activities and deviations from normal behaviour.
- Automated incident response using AI technologies proactively detects, analyses, and responds to security incidents in real-time.
AI is being increasingly used in cybersecurity to improve threat detection, automate incident response, detect anomalies, strengthen authentication processes, and enhance security analytics. AI algorithms can analyse vast amounts of data to identify patterns and anomalies, while machine learning models can detect known and unknown threats. AI systems can also automate incident response by isolating compromised systems, blocking malicious traffic, and containing the impact of cyber attacks. AI technologies can enhance user authentication by verifying identities based on unique characteristics or behavioural patterns. AI-driven security analytics platforms can process large volumes of data in real-time, enabling proactive threat mitigation and strategic decision-making.
Threat detection
AI algorithms, including machine learning, deep learning, and natural language processing, are used in threat detection to analyse data to identify potential security threats. These algorithms analyse large volumes of data, including network logs, user behaviour, and system activities, to identify suspicious activities, anomalies, and indicators of compromise. Machine learning plays a crucial role in detecting patterns and anomalies in cybersecurity data by learning from historical patterns and identifying deviations from the norm.
AI models can detect known threats based on predefined patterns and signatures, enabling the automated identification of common threats like malware, phishing, and unauthorised access attempts. AI also plays a crucial role in identifying unknown threats through anomaly detection and behaviour analysis, monitoring deviations from normal behaviour, and identifying suspicious activities.
Anomaly detection
Anomaly detection is a critical aspect of cybersecurity, focusing on identifying abnormal or suspicious activities within a network or system. AI systems play a crucial role in anomaly detection by leveraging advanced algorithms to analyse vast amounts of data and detect deviations from normal behavior. They can detect various types of anomalies, including network intrusions, unauthorised access attempts, unusual data transfers, and abnormal system behaviours.
AI tools and techniques are used to monitor network traffic and user activity in real-time, providing valuable insights into normal behaviour patterns and potential security threats. By analysing historical data and normal patterns, AI systems can establish baseline behaviour for comparison and detect deviations from normal behaviour through statistical analysis and machine learning algorithms.
Also read: How can generative AI be used in cybersecurity?
AI systems also generate alerts and notifications to inform security teams about potential anomalies for further investigation. This enhances the efficiency and effectiveness of anomaly detection by automating the process of analysing large volumes of data and identifying potential threats. Automation reduces response times to security incidents and minimises the impact of cyber threats. AI systems can also detect sophisticated and stealthy cyber threats that may go unnoticed by traditional security measures.
Automated incident response
Automated incident response is a proactive approach to cybersecurity that uses AI and machine learning technologies to detect, analyse, and respond to security incidents in real-time. This proactive approach helps organisations mitigate the impact of security breaches and reduce response times. Automated incident response systems consist of key components such as threat detection algorithms, response playbooks, orchestration tools, and automated remediation capabilities.
Benefits of automated incident response include reducing manual intervention, accelerating response times, minimising human errors, and enabling organisations to respond more efficiently. AI-powered threat detection plays a crucial role in this process by continuously monitoring network activities, analysing data for anomalies, and identifying potential security threats.
User authentication
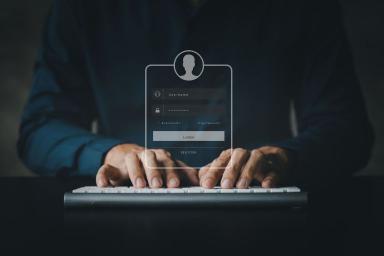
User authentication is a crucial security measure that verifies the identity of users accessing systems, applications, and data, ensuring only authorised individuals can access sensitive information and resources. Key principles of user authentication include something you know (passwords, PINs), something you have (smart cards, tokens), and something you are (biometrics). Multi-factor authentication combines these principles to enhance security and reduce the risk of unauthorised access.
User authentication plays a critical role in access control by determining the level of access rights granted to users based on their verified identity. There are three types of user authentication methods: password-based, multi-factor authentication, and biometric authentication.
AI and machine learning technologies enhance user authentication by analysing user behaviour, detecting anomalies, and identifying potential security threats. Behavioural biometrics offer a non-intrusive and continuous authentication method by creating a unique user profile based on ehavior. Risk-based authentication using AI uses AI algorithms to assess the risk level of user access attempts based on various factors, adjusting authentication requirements based on risk scores.
Security analytics
Security analytics is a crucial process in cybersecurity that uses advanced analytics techniques, machine learning algorithms, and AI technologies to detect and respond to security threats. The primary objectives of security analytics include proactive threat detection, incident response optimization, security posture assessment, and risk mitigation. Key components of security analytics include log analysis, network traffic analysis, endpoint detection and response (EDR), threat intelligence integration, and security information and event management (SIEM) systems.
Also read: AI ‘worm’ raises alarm on cybersecurity vulnerabilities
Machine learning plays a significant role in security analytics by leveraging it for threat detection, behavioral analytics for anomaly detection, and predictive analytics for risk assessment. Threat intelligence sharing and collaboration enable organizations to exchange information on cyber threats, indicators of compromise, and best practices for threat mitigation.Operationalizing security analytics processes involves integrating analytics tools and processes into day-to-day security operations to improve threat detection and incident response.
Phishing detection
Phishing attacks are social engineering tactics used by cybercriminals to deceive individuals into disclosing sensitive information. Common types include email phishing, spear phishing, vishing (voice phishing), smishing (SMS phishing), and pharming. These attacks exploit human vulnerabilities and trust to steal confidential information or install malware on victims’ devices. The impact of phishing attacks can be severe, including financial loss, data breaches, identity theft, and reputational damage.
To detect phishing, email filtering and analysis are essential techniques. Email filtering tools analyze incoming emails for suspicious content, attachments, and links, while URL scanning and link analysis check the legitimacy of URLs to identify malicious websites used in phishing attacks. Phishing simulation and awareness training educate users on recognizing phishing attempts and responding appropriately.
AI technologies, such as machine learning and natural language processing, enhance phishing detection by analyzing email content, sender behavior, and user interactions to identify suspicious patterns. Behavioral analytics use AI to analyze user behavior and communication patterns to detect anomalies that may indicate phishing attempts. Anomaly detection algorithms powered by AI can identify unusual patterns in email communications in real-time, proactively mitigating phishing risks.
Best practices for phishing detection include implementing multi-layered security controls, enabling two-factor authentication (2FA), conducting regular phishing assessments, and practicing incident response procedures. These measures help strengthen defenses and mitigate the impact of successful phishing attacks.