- AI hardware refers to specialised computer hardware designed to perform AI-related tasks efficiently, this includes specific chips and integrated circuits that provide faster processing and energy-saving capabilities.
- Edge computing chips, quantum hardware, application-specific integrated circuits (ASICs), neuromorphic hardware, and field-programmable gate arrays (FPGAs) – all different types of AI hardware – play a critical role in enhancing the capabilities, efficiency, and application potential of AI, driving innovation across a wide range of industries.
- AI hardware faces substantial challenges related to heat management, latency reduction, infrastructure compatibility, quantum computing complexities, security, and ethical considerations, necessitating innovative solutions and collaborative efforts across multiple sectors to ensure its effective and responsible deployment.
The role of AI hardware in machine learning is crucial, as it helps in executing the complex procedures of deep learning models, which can accelerate many processes and drastically reduce the time and cost required for the training and execution of AI algorithms.
Also read: What is Edge AI?
What is AI hardware?
AI hardware refers to the specialised computational devices and components specifically designed and optimised to accelerate AI-related tasks. Unlike general-purpose processors like CPUs (central processing units), AI hardware includes a variety of components such as GPUs (graphics processing units), TPUs (tensor processing units), FPGAs (field-programmable gate arrays), and ASICs (application-specific integrated circuits), each tailored for different types of AI workloads.
Also read: What is voice assistant AI?
Types of AI hardware
1. Edge computing chips
These specialised processors are specifically designed to run AI models at the edge of the network. Using edge computing chips, users can process data and perform critical analytical operations directly near the data source, thus eliminating the need to transfer data to a centralised system.
Edge computing chips have applications in self-driving cars, facial recognition systems, smart cameras, drones, portable medical devices and other real-time decision-making scenarios.
Edge computing chips significantly reduce latency and improve the overall performance of the AI ecosystem by processing data near the data source. In addition, edge computing enhances security by minimising the amount of data that needs to be transferred to the cloud platform.
The following are some of the leading AI hardware manufacturers in the edge computing chip space: Jetson Xavier NX, AMD EPYC™ Embedded 3000 Series, ARM Cortex-M55, and ARM Ethos-U55.
2. Quantum hardware
Quantum computing is a real and advanced computing system that operates on the principles of quantum mechanics. While traditional computers use bits, quantum computing utilises quantum bits to perform calculations.
These quantum bits enable quantum computing systems to process large data sets more efficiently, making them ideally suited for use in artificial intelligence, machine learning and deep learning models.
In drug discovery, quantum hardware can simulate the behaviour of molecules to help researchers accurately identify new drugs. Similarly, in materials science, it could help predict climate change. The financial sector can benefit from quantum hardware by developing price prediction tools.
Quantum computers are much faster than conventional computers and can solve complex problems in seconds that would take billions of years to solve. Quantum computing enables AI models to be trained using large amounts of data in a much shorter time, thus improving the accuracy of predictions and analyses. Quantum computing hardware opens up possibilities for new developments and breakthroughs in the market, unlocking previously unattainable computing power.
3. Application-specific integrated circuits (ASICs)
Application Specific Integrated Circuits (ASICs) are designed for targeted tasks such as image processing and speech recognition. Their purpose is to accelerate the running of AI programs to meet the specific needs of an enterprise business, provide efficient infrastructure, and increase overall speed within the ecosystem.
ASICs are cost-effective compared to traditional CPUs or GPUs. This is due to their power efficiency and superior task performance over CPUs and GPUs.
These integrated circuits can process large amounts of data, making them instrumental in training AI models. Their application areas include natural language processing of text and speech data. Moreover, they simplify the deployment of complex machine-learning mechanisms.
4. Neuromorphic hardware
Neuromorphic hardware represents a significant advancement in computer hardware technology and aims to mimic the functions of the human brain. This innovative hardware mimics the human nervous system, employing a neural network infrastructure that operates in a bottom-up fashion. This network consists of interconnected processors called neurons.
Neuromorphic hardware excels at parallel processing compared to traditional computing hardware that processes data sequentially. This parallel processing capability allows neural networks to perform multiple tasks simultaneously, resulting in increased speed and energy efficiency.
Neuromorphic hardware can be trained with a wide range of datasets, making it suitable for a wide range of applications, including image detection, speech recognition, and natural language processing. Additionally, the accuracy of neuromorphic hardware is phenomenal, as it can learn quickly from large amounts of data.
Self-driving cars can use neuromorphic computing hardware to enhance their ability to sense and interpret their surroundings. In medical diagnostics, neuromorphic hardware can provide image detection capabilities to help identify diseases. Various IoT devices can use neuromorphic hardware to collect and analyse data for efficient data processing and decision-making.
5. Field programmable gate arrays (FPGAs)
Field programmable gate arrays (FPGAs) are advanced integrated circuits. These dedicated chips can be customised and programmed to meet the specific requirements of the AI ecosystem.
FPGAs consist of configurable logic blocks (CLBs) that are interconnected and programmable. This inherent flexibility supports a wide range of applications in artificial intelligence.
Working like read-only memory chips but with higher gate capacity, FPGAs have the advantage of being reprogrammable. This means they can be programmed multiple times, allowing them to be adapted and scaled to changing requirements. FPGAs are more efficient than traditional computing hardware, providing a powerful and cost-effective architecture for AI applications.
In addition to customisation and performance benefits, FPGAs offer enhanced security measures. Their complete architecture ensures robust protection, allowing them to reliably enable safe AI.
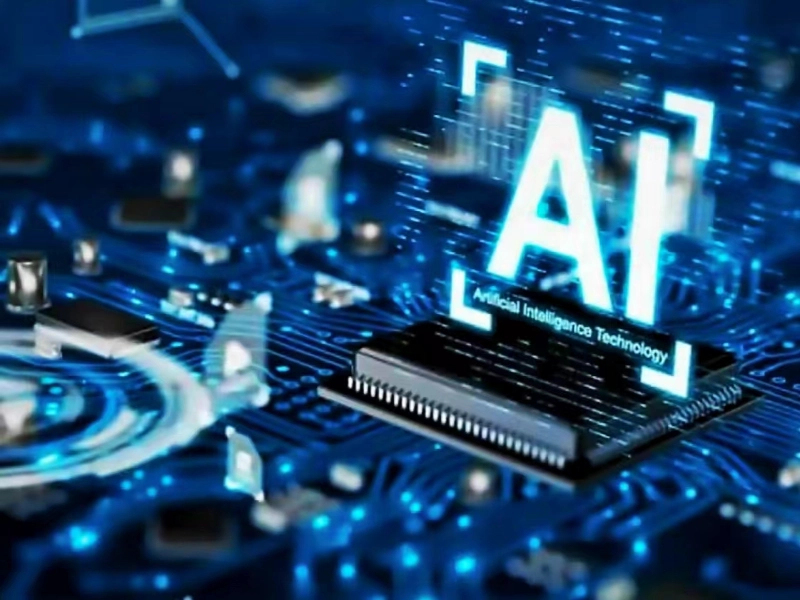
Challenges facing AI hardware
AI hardware, particularly high-performance GPUs, ASICs, and quantum computers, generates significant heat during operation. Managing this heat and ensuring efficient power consumption are critical challenges. Effective cooling systems and energy-efficient designs are necessary to prevent overheating and reduce energy costs, which can be substantial in large-scale AI operations.
For edge computing chips, minimizing latency is crucial, especially in real-time applications like autonomous vehicles and smart cameras. Ensuring that data is processed quickly and efficiently near the data source without delays is a persistent challenge.
AI hardware must be compatible with existing infrastructure. Integrating new AI hardware with legacy systems can be complex, requiring extensive modifications and optimizations to ensure seamless operation.
Quantum computing hardware faces unique challenges, including maintaining qubit coherence, error rates, and the need for extremely low temperatures for superconducting qubits. These technical hurdles make the development and deployment of quantum hardware particularly challenging.
AI hardware must ensure robust security measures to protect sensitive data and prevent unauthorized access. The integration of AI hardware into critical applications, such as healthcare and finance, necessitates stringent security protocols to safeguard data privacy and integrity.
AI hardware that enables advanced AI capabilities raises ethical concerns, particularly regarding privacy, surveillance, and bias. Ensuring that AI hardware is used ethically and responsibly is an ongoing challenge that requires cooperation between manufacturers, regulators, and end-users.