- AI automation refers to the use of artificial intelligence technology to enable machines to perform various tasks and processes autonomously. This includes automated production lines, automated customer service, automated data analysis and decision-making.
- AI automation leverages advanced technologies like machine learning, deep learning, natural language processing, and machine vision to enhance and automate processes across various domains, improving efficiency, accuracy, and decision-making capabilities.
- AI automation is transforming industries such as manufacturing, customer service, financial services, healthcare, and retail by optimising processes, enhancing efficiency, and providing personalised experiences.
AI automation (artificial intelligence automation) refers to the use of AI technologies and algorithms to automate a wide range of tasks and processes to increase efficiency, reduce costs, and reduce the need for human intervention. This technology is used in a wide range of industries and sectors, from manufacturing to customer service to finance and healthcare. This blog will detail the concepts, principles and applications of AI automation.
Basic concepts
AI automation combines AI technologies and other tools to automate business processes. This automation can occur through software—in which AI systems analyse data, learn from it, and make decisions—or through hardware, such as robotic process automation (RPA) in the physical world.
AI automation uses AI techniques like machine learning algorithms, natural language processing (NLP), and computer vision to process and learn from large amounts of data. Once an AI application processes that data and builds an AI model, it can direct intelligent decision-making based on what it learned.
Also read: 2023 Ultimate Artificial Intelligence And Automation Developer Bundle Unveiled
Principles and technologies
Machine learning is one of the core technologies of AI automation. It enables computer systems to learn from data and incrementally improve their performance without being explicitly programmed. The main machine learning techniques include supervised learning, unsupervised learning and reinforcement learning.
Supervised learning refers to training with labelled datasets to predict the output of new data. Common algorithms include linear regression, decision trees, and neural networks.
Unsupervised learning is training on unlabelled datasets to discover patterns and structure in the data. Common algorithms include clustering and dimensionality reduction.
Reinforcement learning refers to learning through a process of trial and error, adjusting strategies to maximise rewards based on the outcome of actions. This approach is particularly useful in automated control and decision-making.
Deep learning is a branch of machine learning that mimics the structure and function of the human brain to learn and process complex data and tasks through deep neural networks. Deep learning has achieved great success in areas such as speech recognition, image recognition, and natural language processing.
Natural language processing enables computers to understand, analyse and generate natural language text. NLP techniques are widely used in tasks such as text classification, sentiment analysis and machine translation.
Machine vision enables computers to understand and interpret image and video data. It includes technologies such as image recognition, target detection and face recognition. It is widely used in automated driving, medical image analysis, security monitoring and other fields.
Also read: Deep learning in computer vision: Revolutionising AI applications
Automated decision-making systems use AI technology to analyse data and make decisions, thereby automating the decision-making process. Such systems play an important role in financial transactions, risk management and supply chain optimisation.
An automated rule engine is a rule-based system that uses predefined rules to automate specific tasks and processes. Such systems are typically used in business process automation and decision support.
Data-driven automation uses big data and data analytics to automate decision-making and process optimisation. By collecting, analysing and utilising large amounts of data, smarter and more efficient automation systems can be achieved.
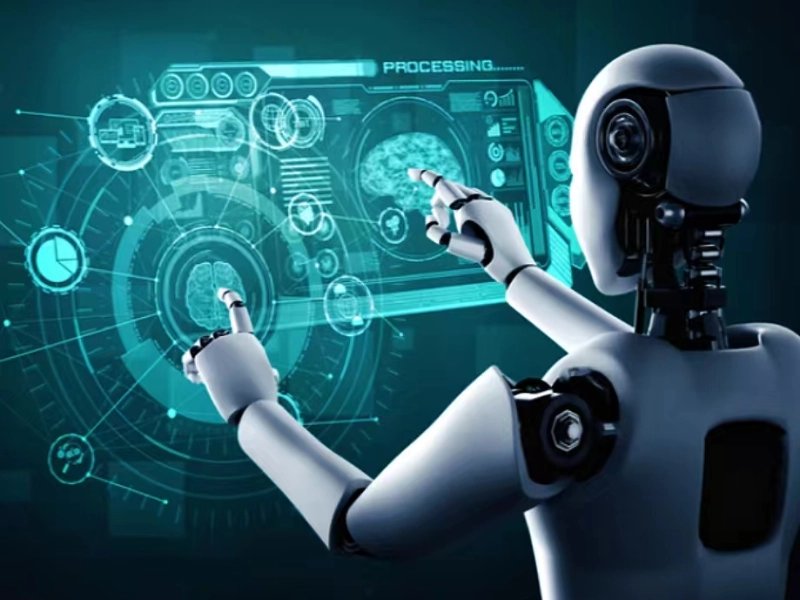
Application areas
1. Manufacturing
AI automation in manufacturing aims to optimise production processes and improve productivity and quality. Among the specific applications are smart manufacturing and predictive maintenance.
Intelligent manufacturing covers automated production lines and robotic manufacturing, which utilise machine learning and machine vision technologies to achieve intelligent monitoring and scheduling, thereby improving production efficiency and product quality.
Predictive maintenance uses machine learning technology to monitor and predict the operating status of equipment and machines, which can detect and prevent equipment failures in advance, thus reducing production line downtime and maintenance costs.
Tesla‘s Gigafactory employs AI automation to optimise its manufacturing processes for electric vehicles (EVs) and batteries. Robots equipped with Robots equipped with computer vision systems automate assembly tasks, ensuring precision and efficiency in production.
2. Customer service
AI automation can be used to provide personalised and efficient services to improve customer satisfaction. Specific applications include virtual assistants and intelligent recommendations.
Virtual assistant is the use of natural language processing and machine learning technology to develop virtual assistants and intelligent customer service systems to provide customers with 24/7 online support and answers.
Intelligent recommendation refers to the use of machine learning technology to achieve personalised product recommendation and service promotion based on user behaviour and preference data, improving sales and customer conversion rates.
3. Financial services
AI automation can be used to automate trading and risk management to improve trading efficiency and accuracy.
Quantitative trading using machine learning and algorithmic trading technology, automated trading decisions based on market data and model predictions, improving trading efficiency and profitability.
It can also conduct real-time monitoring and analysis of user behaviour and transaction data to identify and prevent fraud and reduce financial risks.
4. Healthcare
AI automation can be used to improve the diagnosis and treatment process and enhance the quality and efficiency of healthcare services.
For example, the use of machine learning and machine vision technology to automate the analysis and diagnosis of medical image data, assisting doctors in disease diagnosis and treatment planning.
At the same time, based on the patient’s genetic data and medical history information, machine learning technology is used to achieve personalised treatment plans and drug recommendations, improving treatment results and patient satisfaction.
IBM Watson for Oncology is an AI-powered platform that assists healthcare professionals in cancer diagnosis and treatment planning. By analysing patient data, medical literature, and treatment guidelines, Watson provides personalised treatment recommendations, helping oncologists make informed decisions and improve patient outcomes.
5. Retail
AI automation can be used to optimise inventory management and sales strategies to improve sales and customer experience. Specific applications include intelligent inventory management and intelligent recommendation systems.
Intelligent inventory management is the use of machine learning technology to analyse and forecast sales data and inventory data, optimise inventory management and replenishment strategies, and reduce inventory backlogs and stock-outs.
An intelligent recommendation system refers to the use of machine learning technology to achieve personalised product recommendations and shopping guidance based on users’ purchase history and preference data, improving sales conversion rate and customer satisfaction.