- Data security measures in AI systems include encryption techniques, access controls, secure data storage practices, data masking, and anonymization to safeguard sensitive information and comply with data protection regulations.
- Building robust AI models is crucial to detecting and mitigating adversarial attacks, ensuring reliability, trustworthiness, system integrity, and ethical considerations in AI systems.
- Privacy protection in AI applications involves confidentiality, user consent, minimising data collection, and implementing privacy-preserving techniques like differential privacy, federated learning, and homomorphic encryption.
AI security is the protection of artificial intelligence systems and technologies from cybersecurity threats and vulnerabilities. It involves data security, model robustness, privacy protection, bias and fairness, accountability and transparency, and continuous monitoring and updates. Data security involves implementing encryption, access controls, and secure storage practices to safeguard sensitive information. Model robustness ensures AI models are resilient to adversarial attacks, while privacy protection protects user privacy. Bias and fairness address biases in AI algorithms, while transparency measures increase trust in AI technologies. Continuous monitoring and updates ensure ongoing security and prevent potential breaches.
Data security in AI systems
Data security is crucial in AI systems, as it is the foundation for training algorithms and making decisions. However, data is often sensitive, and unauthorised access can lead to privacy violations, identity theft, financial losses, and reputational damage. To ensure data security, organisations should implement encryption techniques, set up access controls, secure data storage practices, use data masking and anonymization techniques, and establish data governance policies.
Various encryption techniques are available for data security in AI systems, including symmetric encryption, asymmetric encryption, homomorphic encryption, end-to-end encryption, and data tokenization. These techniques ensure confidentiality, integrity, and compliance with data protection regulations, retention policies, and sharing practices. By implementing robust data security measures, organisations can safeguard sensitive information, mitigate data breaches, and build trust with users regarding data privacy and security. Data security is also a critical aspect of AI governance and compliance, ensuring that AI applications adhere to regulatory requirements and ethical standards in data handling and processing.
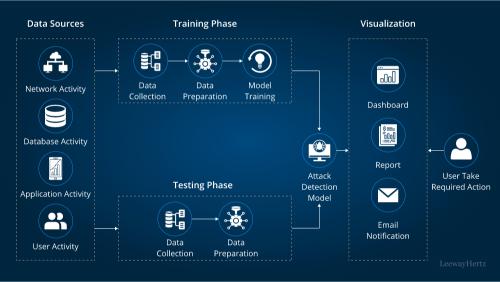
Ensuring model robustness
Adversarial attacks are deliberate attempts to manipulate AI models by introducing subtle changes to input data. They can lead to incorrect predictions, compromised security, and biassed decision-making in AI systems. Building robust AI models is crucial for their reliability, trustworthiness, system integrity, and ethical considerations. Techniques for detecting and mitigating adversarial attacks include adversarial training, robust optimisation, defensive distillation, model interpretability, and adversarial detection mechanisms.
Real-world applications of robust AI models include cybersecurity, autonomous vehicles, and healthcare. Cybersecurity defences can be enhanced by detecting and mitigating cyber threats, while autonomous vehicles can ensure safety and reliability. Healthcare systems can protect patient data and medical AI systems from adversarial attacks, maintaining the accuracy and integrity of diagnoses and treatment recommendations.
By prioritising the development of robust AI models and implementing techniques to detect and mitigate adversarial attacks, organisations can enhance the security, reliability, and trustworthiness of their AI systems. Ensuring model robustness is essential for mitigating risks, maintaining ethical standards, and fostering a secure and resilient AI ecosystem in various industries and applications.
Privacy protection in AI
Privacy protection in AI applications is crucial for maintaining trust and compliance with data protection regulations. It involves ensuring the confidentiality and integrity of user data, obtaining user consent for data collection and processing, and minimising data collection to only what is necessary for AI tasks. Adhering to GDPR requirements, CCPA regulations, and industry-specific regulations is essential for data privacy and consumer rights.
Privacy-preserving techniques in AI systems include differential privacy, federated learning, secure multiparty computation, and homomorphic encryption. Ethical considerations in privacy protection include fairness and transparency, bias detection and mitigation, and data anonymization and de-identification.
Also read: US and UK cooperate on AI security and testing
By implementing these measures, organisations can uphold user privacy rights, comply with data protection regulations, and foster trust in AI technologies. Prioritising privacy-preserving techniques and ethical considerations in AI development and deployment is essential for promoting responsible data handling, transparency, and accountability in the use of AI applications across various industries and sectors.
Addressing bias and ensuring fairness
AI algorithms can be influenced by various sources of bias, including data, algorithmic, and societal biases. These biases can lead to unfair outcomes and discriminatory decisions, affecting marginalised groups and undermining trust in AI systems. To detect and mitigate biases, bias detection methods are used, including demographic, selection, and representation bias.
Fairness in AI decision-making is crucial for ethical considerations, legal implications, and user trust. Compliance with anti-discrimination laws and regulations is essential to preventing discriminatory practices. Building trust with users by demonstrating fairness in AI algorithms and promoting transparency is also essential.
Strategies for promoting fairness and reducing bias in AI systems include data preprocessing, fairness-aware algorithms, bias mitigation techniques, fairness audits, and diversity and inclusion initiatives. By addressing bias and ensuring fairness in AI systems, organisations can enhance the ethical and social impact of AI technologies, promote equity and inclusivity, and build trust with users and stakeholders.
Accountability and transparency in AI
Accountability and transparency in AI systems are crucial for ensuring ethical practices, building trust with users and stakeholders, and demonstrating responsible AI governance. This involves holding individuals, organisations, and AI systems accountable for their actions, decisions, and outcomes in compliance with ethical standards and regulatory requirements.
Also read: Revolut’s AI security slashes fraud losses by 30%
Legal implications include compliance with data protection laws, privacy regulations, and industry standards to protect user rights and mitigate risks. Transparency in AI decision-making involves using explainable AI (XAI), model interpretability, and algorithmic transparency to provide transparent explanations for AI decisions.
Establishing accountability frameworks in AI development involves adopting responsible AI principles, implementing governance structures, conducting risk assessments, and implementing accountability mechanisms. Ethical review boards evaluate AI projects, maintain audit trails and documentation, and engage stakeholders to gather feedback and promote transparency and accountability.
Continuous monitoring and updates for AI security
Continuous monitoring and updates are crucial for AI security, as they help organisations identify and respond to evolving cybersecurity threats and vulnerabilities. This includes proactive risk management, ensuring compliance with regulatory standards, and implementing security controls. The process involves real-time monitoring, threat intelligence, log analysis, and security controls. Regular updates and patch management are also essential for addressing known vulnerabilities and enhancing system security.
Version control, a secure development lifecycle, and incident response planning are also crucial. Collaborative efforts, including cross-functional teams, security training, and external partnerships, are essential for establishing shared responsibilities, communication channels, and best practices for security monitoring and updates. These efforts help maintain the security, integrity, and resilience of AI systems in the face of cybersecurity challenges and emerging threats.