- Tech giant Tencent held its Hi Tech Day and 2023 Digital Open Things Conference at the China National Convention Centre in Beijing on 14 December, with the theme “Intelligence emerges and opens up all things”.
- Jiang Chunyu said that China’s AI development urgently needs trainable, high-quality data sets.
- Jiang revealed that a white paper on AI data governance will be released soon to establish a system of methods and rules in this field.
China is accelerating its digital transformation and bridging the digital divide, with strong support for the application of new technologies such as data, cloud computing, artificial intelligence and quantum computing. Tech giant Tencent held Hi Tech Day and 2023 Digital Open Things Conference with the theme of “Intelligence Emerges, Digital Opens All Things” at the China National Convention Center in Beijing on December 14, inviting big names from all walks of life to discuss the trend of artificial intelligence.
At the conference, Jiang Chunyu, director of the Cloud Data and Blockchain Department of the China Academy of Information and Communication Research, gave a speech on the topic of “AI data governance triggers thinking”.
High-quality big database is the next evolutionary goal
“There are not many trainable, high-quality datasets on the market, especially in the Chinese context, where a lot of high-quality data is hidden. We urgently need to pass a marketable, open model, or which model can release the data and can be used by everyone.”
Jiang Chunyu, director of the Cloud Data and Blockchain Department of the China Academy of Information and Communication Research
Since 2018, general AI is leading the wave of technology.All parties have gone all out and invested money in big model training, creating a massive competitive trend. However, Jiang Chunyu believes that domestic development should set its sights on data enhancement, not only in terms of quantity, but also in terms of quality. China as a natural data power, rather than in the algorithm and arithmetic of the gap between these parties is not large, the huge cost of the field “involution, rather than to improve the quality of data, may bring better results.
He listed for the audience the large-scale, diverse, high-quality datasets needed for large model training, GPT-1 four or five years ago required 4.8GB of high-quality data, GPT-2 was 40GB, GPT-3 was 570GB, and this year, Meta launched a large model of the database reached a staggering 4,000GB in size.
Jiang expressed his concern: “There are not many trainable, high-quality datasets on the market, especially in the Chinese context, where a lot of high-quality data is hidden. We urgently need to pass a marketable, open model, or which model can release the data and can be used by everyone.”
Also read: Amazon Q AI assistant: AWS launches a revolutionary data query approach
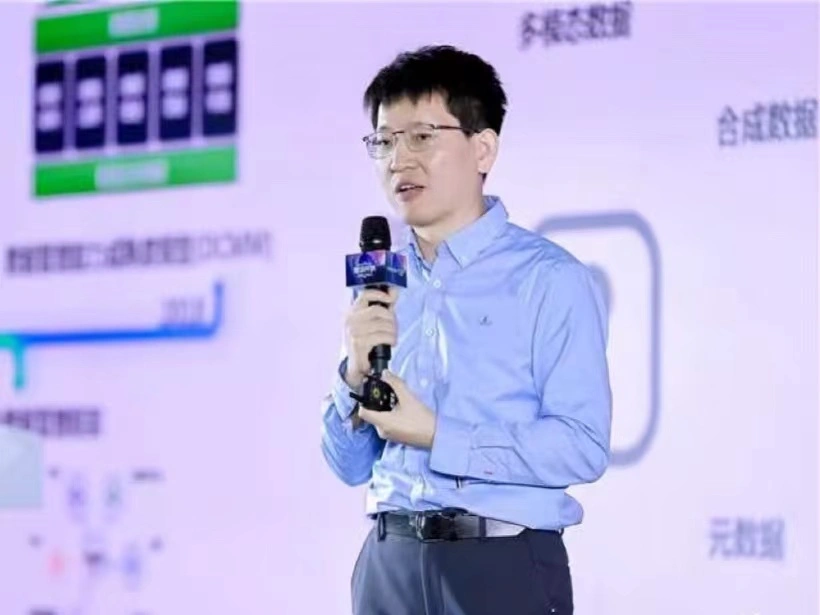
Data management, security and protection system urgently needs to be established
Jiang was present to raise three three problems with the current development of the industry:
- Data quality is generally biased
In order to transform poor quality datasets into high quality, Jiang emphasised the establishment of an integrated system of data engineering system and DevOps R&D operations. From R&D delivery, data operation and maintenance to value operation, a complete data production chain or supply chain is formed so that data can be delivered in an orderly manner and gradually strung together to form a production pipeline, which is different from the traditional structured data processing in the past.
He also warned the companies in the room not to invest a lot of time in model training before improving data quality is complete, a training may cost tens of millions of dollars but to no avail. Surprisingly, their team is sorting out the methodology and framework of AI training methods, completing a white paper on artificial intelligence data governance, and establishing a system of methods and rules in this area.
- Security and privacy issues
Jiangsaid that, “There are a large number of security issues and privacy issues involved in the whole training process, including enforcement rights, personal information collection violations, insecure data transmission, data information tampering, and insecure storage and transmission of models. In addition, there are also problems such as Prompt attacks and generated content violations.
In order to ensure full lifecycle privacy protection and security protection in the production, use and operation of models, we need to master a variety of technologies, establish appropriate rules, and configure the capabilities of auditors and monitors as a whole. This is an entirely new area that requires attention and investment to meet the evolving data security and privacy challenges.”
- Management of generated and synthetic content
Even synthetic data cannot be a fraud. Therefore, measurement of truthfulness and accuracy is particularly critical.
On top of this, detection and prevention of harmfulness is also an urgent task. Currently, many large-scale models are reported precisely because of problems in the generated content, such as harassment, violence, and discrimination. These issues must be effectively controlled.
Separately, the requirements for authenticity and accuracy can be constrained by rules; the requirements for content generation, monitoring mechanisms, and authenticity assessment can be realised through automatic detection of content identification and filtering combined with manual auditing; and the prevention of harmfulness issues can be effectively managed through the use of constraints on rules, line prediction, empirical privacy assessment, and privacy attack testing.