- In the age of AI, privacy has become an increasingly complex issue. With the vast amount of data being collected and analysed by companies and governments, individuals’ private information is at greater risk than ever before.
- The ethical development of AI is central to maintaining public trust, while the global nature of data privacy laws presents significant challenges for cross-border AI operations.
- Consumer awareness around privacy is growing, but there is still a significant gap between the control users want and the power they currently have over their data.
OUR TAKE
As technology continues to advance at an unprecedented pace, artificial intelligence (AI) is becoming increasingly embedded in various aspects of our daily lives. From generative AI capable of producing any content from a simple prompt to smart home devices that adapt to our habits and preferences, AI holds the potential to transform how we engage with technology.However, as the amount of data we generate and share online grows exponentially, concerns surrounding privacy have become more critical than ever.
-Tacy Ding, BTW reporter
In recent years, several high-profile incidents have highlighted the concerning intersection of AI technology and privacy. The Cambridge Analytica scandal in 2016 exposed how the firm harvested data from 87 million Facebook users for political advertising without consent, prompting global outrage and substantial penalties for the platform. Clearview AI’s emergence in 2017 raised alarms as it created a vast facial recognition database by scraping publicly available images from social media, leading to legal scrutiny in multiple countries. Additionally, Amazon’s Ring faced backlash for allowing police access to users’ camera footage, which sparked debates about surveillance and privacy. In 2019, Google Nest cameras were reported to have shared user videos without notification, eroding trust in the company’s privacy practices. Most recently, in 2023, Meta agreed to pay $1.4 billion to settle a landmark privacy case in Texas, underscoring the growing scrutiny on tech companies regarding data protection and user rights. These cases collectively illustrate the urgent need for robust privacy safeguards in the age of AI.
Also read: What is artificial intelligence?
Also read: Meta’s $1.4B payout in landmark Texas privacy case
Importance of privacy in the digital era
Privacy is the right to maintain the confidentiality of personal information and protect it from unauthorised access. It is a fundamental human right that grants individuals control over their personal data and its usage. Today, privacy is more crucial than ever, as the volume of personal data collected and analysed continues to increase.
Firstly, it safeguards individuals from harm, such as identity theft or fraud. It also preserves individual autonomy and control over personal information, which is vital for personal dignity and respect. Moreover, privacy enables individuals to sustain their personal and professional relationships without the fear of surveillance or interference. Finally, it protects our free will; if all our data is publicly accessible, harmful recommendation algorithms could analyse our information and manipulate individuals into making specific (purchasing) decisions.
As artificial intelligence (AI) increasingly permeates all aspects of modern life, from healthcare and education to finance and entertainment, the question of how to ensure that AI systems respect user privacy has never been more urgent. AI’s potential is immense, and its growing intelligence has helped solve some of the most complex challenges across industries. However, the very intelligence that makes AI so valuable relies on one core ingredient: data. And therein lies the dilemma—can we harness AI’s power while also safeguarding privacy?
Also read: Oracle settles $115M privacy lawsuit over data collection
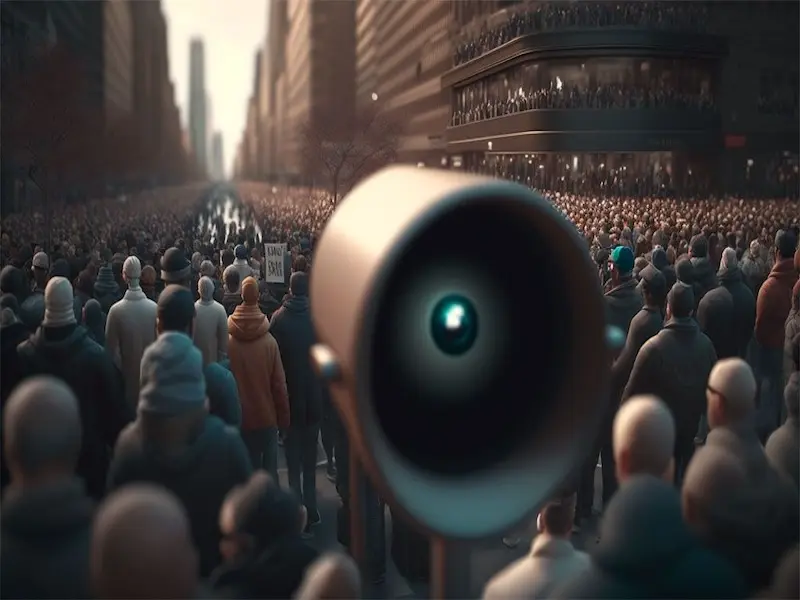
Privacy legislation: Guardrails for AI?
The growing concerns surrounding AI and privacy have spurred governments around the world to take action. In 2018, the European Union implemented the General Data Protection Regulation (GDPR), a comprehensive law designed to give individuals more control over their personal data and hold companies accountable for how they collect, store, and use that data. Under GDPR, individuals must provide explicit consent for their data to be collected, and companies are required to demonstrate transparency in their data-handling practices.
GDPR has significantly influenced the development of AI systems within the EU, particularly around the principle of data minimisation. AI developers are now legally required to limit the amount of personal data they collect and use only what is necessary for a specific task. This has prompted some AI researchers and companies to explore alternatives to data-heavy AI models, focusing on privacy-preserving technologies that can mitigate the risk of privacy violations.
Outside the EU, privacy regulations are less uniform. The United States, for instance, lacks a single federal privacy law comparable to GDPR, although states such as California have introduced their own comprehensive data protection laws, including the California Consumer Privacy Act (CCPA). The CCPA gives consumers more control over their personal information, allowing them to opt-out of data collection practices or request that their data be deleted.
In Asia, countries like Japan and South Korea have also revised their privacy laws to address AI-related concerns. Japan’s Act on the Protection of Personal Information (APPI) has been updated to align more closely with GDPR, reflecting the growing recognition that privacy must be central to AI development. South Korea’s Personal Information Protection Act (PIPA) is another example of robust privacy legislation that governs data handling in AI systems.
The role of privacy-enhancing technologies
While regulations such as GDPR set important guardrails for AI, they do not solve the inherent conflict between AI’s need for data and the desire for privacy. To address this, a growing field of research is focused on developing privacy-enhancing technologies (PETs) that aim to make AI systems both smart and secure. These technologies enable AI systems to function without compromising user privacy, offering a potential solution to the privacy dilemma.
Differential privacy: is one of the most promising PETs. It works by adding random noise to datasets in a way that protects individual data points while still allowing AI to learn from the overall data patterns. This allows AI systems to generate accurate insights without revealing sensitive information about specific individuals.
Federated learning: is another approach that addresses privacy concerns. Instead of sending raw data to a central server for processing, federated learning allows AI models to be trained across multiple devices, with each device processing its own local data. Only the aggregated model updates are shared, which reduces the need to centralise personal data and thus minimises privacy risks.
Homomorphic encryption: takes a more advanced approach, enabling AI systems to process encrypted data without ever needing to decrypt it. This ensures that sensitive data remains protected throughout the entire computation process, eliminating the risk of exposing personal information even during analysis.
While these technologies show promise, they are not without challenges. Differential privacy can reduce the accuracy of AI models, particularly in cases where highly precise predictions are needed. Federated learning, on the other hand, requires significant computational resources, and ensuring the security of model updates remains a challenge. Homomorphic encryption, though highly secure, can be computationally expensive and slower than traditional methods.
Which of the following is not a technology of privacy-enhancing?
A. Differential privacy
B. Homomorphic encryption
C. Deep learning
D. Federated learning
The correct answer is at the bottom of the article.
The role of ethical AI development
As AI systems become more integrated into everyday life, the need for ethical AI development has gained increasing attention. Beyond regulatory compliance, ethical AI focuses on embedding moral principles, fairness, transparency, and accountability into the entire lifecycle of AI systems—from design to deployment. These ethical considerations address not only privacy but also broader concerns, such as bias, discrimination, and the societal impact of AI.
Ethical AI frameworks
Several companies and institutions have developed ethical AI frameworks aimed at guiding the development and deployment of AI systems. Google, for instance, introduced its AI principles, which emphasise fairness, privacy, and avoiding harmful outcomes. Microsoft has established a similar framework that includes transparency, accountability, and inclusivity. These frameworks are designed to ensure that AI systems are built in ways that respect privacy, minimise harm, and provide accountability when things go wrong.
However, these ethical AI frameworks have faced criticism for being vague or lacking enforcement mechanisms. Critics argue that many ethical guidelines function more as public relations tools rather than actionable policies that guide day-to-day AI development. For instance, some companies have been accused of “ethics washing,” where they publicly commit to ethical principles without implementing substantial changes to their operations or governance.
Balancing privacy with AI’s ethical goals
In the context of privacy, ethical AI development involves critical decisions about how much data to collect and how that data is used. While privacy regulations like GDPR offer legal guidance, ethical AI frameworks often push developers to go further. This includes designing AI systems that use privacy-by-design principles, where privacy protection is built into the technology from the outset, rather than being an afterthought. For example, privacy-by-design might involve minimising data collection to only what’s necessary for the AI to perform its function or implementing strong anonymisation techniques.
Furthermore, ethical AI principles encourage transparency. This means providing users with clear information about how their data will be used by AI systems and offering easy-to-understand consent mechanisms. Transparency also involves making the AI system’s decisions explainable, ensuring that users understand how and why AI arrived at certain conclusions, especially in sensitive areas such as healthcare or hiring decisions.
Ethical AI in practice: Challenges and trade-offs
Despite the focus on ethical AI, there are significant challenges in implementing these principles. One major issue is the tension between ethical ideals and business objectives. For example, companies may be incentivised to collect more data to improve their AI models or increase personalisation for advertising, even though this conflicts with the principle of data minimisation.
Additionally, embedding ethics into AI development requires a multidisciplinary approach, combining expertise in technology, law, philosophy, and social science. This is not always feasible, as many AI projects are driven by tight deadlines and commercial pressures. To overcome these barriers, some companies have established dedicated AI ethics boards. However, the effectiveness of these boards varies, and they often lack real power to enforce decisions, especially when those decisions conflict with business goals.
Ultimately, ethical AI development is about fostering trust—trust between developers, users, and the wider public. If AI systems are to succeed without infringing on privacy, companies must demonstrate a genuine commitment to ethical principles and ensure that these principles are not just guidelines, but actionable policies with meaningful oversight.
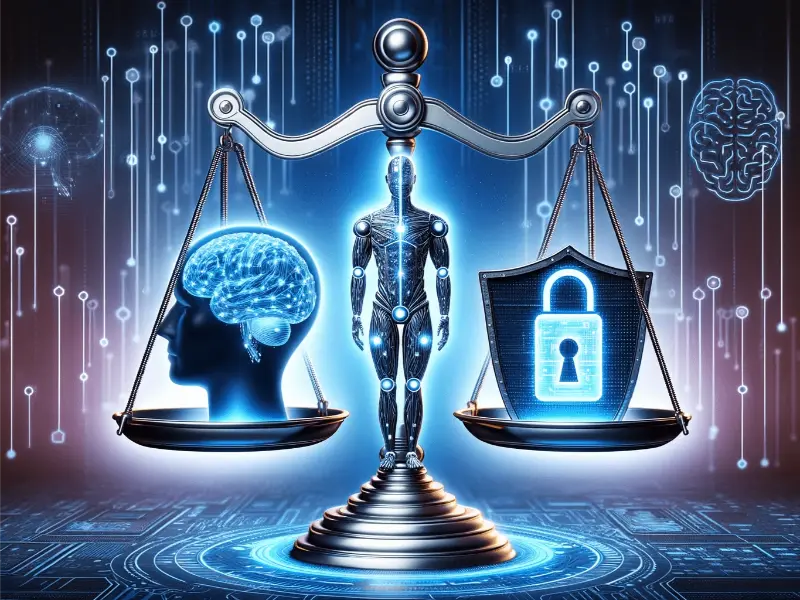
Consumer awareness and choice: Empowering users in the AI age
As AI systems become more embedded in daily life, consumer awareness around privacy has significantly increased. High-profile data breaches, such as the Cambridge Analytica scandal and numerous cyberattacks on major corporations, have made consumers more conscious of the potential risks associated with AI and data privacy. This rising awareness has triggered a demand for greater transparency and control over personal data, placing additional pressure on companies to prioritise privacy and inform users about how their data is being collected and used by AI systems.
Privacy is one of the top issues of the century. We’re at a point where we must decide how much data is appropriate to collect, and how that data should be used.
Tim Cook, CEO of Apple
Transparency and informed consent
One of the primary ways companies have responded to the demand for more consumer control is through transparency initiatives, particularly in relation to data collection. Today, most websites and apps feature pop-ups requesting user consent for cookies or data processing. These consent mechanisms are intended to comply with regulations like the GDPR and the California Consumer Privacy Act (CCPA), which mandate that consumers be given the choice to opt out of data collection or processing.
However, transparency often falls short in practice. Many consent forms are filled with legal jargon or are designed in a way that encourages users to “agree” without fully understanding the implications. This practice, known as dark patterns, refers to design strategies that subtly coerce users into making choices that favour the company’s interests, such as consenting to extensive data collection or agreeing to targeted advertising. Despite the availability of consent options, many users feel disempowered by the complexity of privacy notices, leading to a sense of resignation and mistrust.
In addition, terms of service agreements (TOS), which outline how personal data is handled, are often long and difficult to understand, causing most users to accept them without reading. Research has shown that consumers rarely read the fine print when signing up for services, even though they are legally agreeing to terms that may involve extensive data sharing. This raises the question of whether consent can truly be considered “informed” when users lack the time or understanding to fully grasp what they are agreeing to.
Giving consumers more control: Privacy tools
In response to growing consumer demand for privacy, companies have introduced a variety of privacy tools that allow users to take more control over their personal data. For example, Google and Facebook provide privacy dashboards where users can manage their data, see what has been collected, and adjust their settings for things like location tracking or personalised ads. These tools empower users to decide how much data they want to share and with whom.
Additionally, privacy-focused products like virtual private networks (VPNs) and encrypted messaging apps (such as Signal or WhatsApp) have gained popularity as consumers seek ways to protect their digital communications from surveillance. These services allow users to maintain more control over their online activities by shielding their data from being easily accessed by third parties.
Apple has also led the way in offering more robust privacy controls for its users. With iOS 14, Apple introduced an App Tracking Transparency feature, which requires apps to explicitly request permission before tracking users across other apps and websites. This initiative, which has caused friction with companies like Facebook that rely on targeted advertising, reflects a growing trend of companies positioning themselves as champions of privacy.
The rise of privacy-conscious brands
In response to growing privacy concerns, some companies are now marketing privacy as a key feature. Apple, for instance, has positioned itself as a leader in privacy, highlighting its commitment to keeping user data secure and promoting features like encrypted messaging, limited data tracking, and offline Siri processing. By framing privacy as a selling point, companies are beginning to differentiate themselves in a marketplace where consumers are becoming more selective about which brands they trust with their data.
This shift toward privacy as a competitive advantage reflects the changing consumer landscape. As more users become aware of the risks associated with data collection, they are more likely to favour companies that offer greater privacy protections. This has led to the growth of privacy-focused startups and platforms, such as DuckDuckGo, a search engine that promises not to track users, or ProtonMail, which provides encrypted email services. These services cater to a growing segment of privacy-conscious consumers who prioritise data security over the convenience of traditional platforms.
Final thoughts
Safeguarding privacy in the AI age is an issue that concerns us all, both as individuals and as members of society. Addressing this challenge requires a multifaceted approach, combining both technological and regulatory measures. Decentralised AI technologies present a promising path forward, offering secure, transparent, and accessible AI services and algorithms. By utilising these platforms, we can mitigate the risks associated with centralised systems while fostering greater democratisation and accessibility of AI solutions.
At the same time, it is crucial for governments and regulatory bodies to take an active role in overseeing the development and deployment of AI technologies. This involves establishing regulations, standards, and oversight mechanisms that ensure the responsible and ethical use of AI while safeguarding individual privacy rights.
Ultimately, protecting privacy in the AI era demands collaboration and cooperation among a wide range of stakeholders, including governments, industries, and civil society. By working together to formulate and implement strategies that prioritise privacy and security, we can help ensure that the benefits of AI are realised in a manner that is ethical, responsible, sustainable, and respectful of the privacy and dignity of all individuals.
The correct answer is C. Deep learning.