- In spatial epidemiology, GeoAI analyses the impact of the environment on health, improving disease tracking and public health response.
- GeoAI uses satellite imagery and geospatial data to streamline mining processes, predict mineral locations and reduce environmental impact.
- Despite its potential, GeoAI requires large, detailed datasets for effective implementation and impactful results.
OUR TAKE
GeoAI opens up new possibilities in spatial epidemiology, enhancing our ability to understand environmental influences on health with unprecedented precision. This enables public health officials to identify and act on environmental factors that affect health outcomes, enabling more targeted interventions. Beyond health, GeoAI’s impact extends to mining and urban planning, optimising operations and promoting sustainability. These diverse applications not only demonstrate the versatility of GeoAI, but also its potential to transform future public health strategies and resource management practices.
–Heidi Luo, BTW reporter
GeoAI is everywhere, and it’s part of our daily lives. On a typical Friday evening, as you leave work and check your phone for traffic information, you’re actually using some pretty sophisticated GeoAI processes. It’s a simple act of checking the traffic, but it’s GeoAI that can help you assess the congestion on the roads, predict peak times, and suggest the best routes to take.
While GeoAI is often thought of as a way to make things easier for us in our everyday lives, like helping to navigate, it can also be used in more important areas. New research shows how it can help us understand more about how our environment affects our health as we age.
According to a recent study published in the journal Health and Place, GeoAI can help us collect more detailed data in these areas, which means we can more accurately assess the impact of our environment on our health over time.
What is GeoAI?
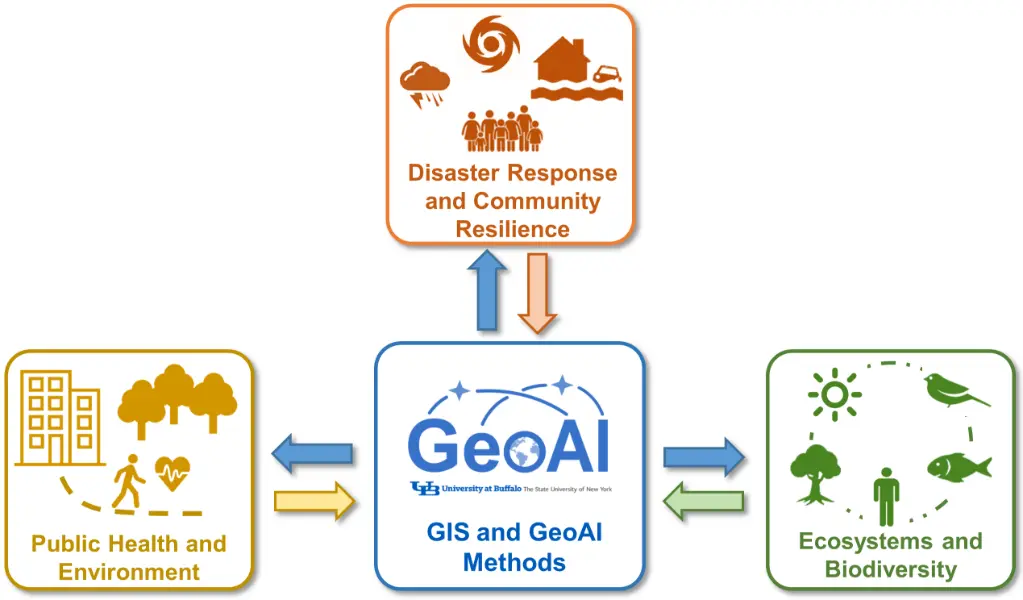
Geospatial Artificial Intelligence (GeoAI) integrates artificial intelligence and machine learning techniques with geospatial data to analyse and interpret information tied to geographic locations. The field uses various types of geospatial data, such as satellite imagery, weather station output, digital maps, and human movement tracking data, to derive spatially-oriented insights.
The uses of GeoAI are many and varied, from spatial epidemiology to healthcare, mining, environmental monitoring, urban planning, resource management, and more. It allows for more sophisticated spatial decision-making and predictive analytics.
Understanding GeoAI in spatial epidemiology
Spatial epidemiology is an important field that looks at how where we live affects our health. It looks at how the environment – such as where people live, work and play – affects their health over time. This study helps us understand how diseases spread, identify the things that make some areas more likely to get sick, and develop targeted public health solutions.
Traditional methods of spatial epidemiology, such as longitudinal studies and space-time regression, have been very helpful in understanding health inequalities influenced by environmental factors. However, these methods have limitations when it comes to handling the huge amounts of data needed to analyse how environmental factors affect people over time. This makes it difficult to really understand the subtle effects of environmental change.
But GeoAI brings together AI and geospatial science to make it easier to analyse complex, multi-scale data. This technology is particularly useful for health inequalities research because it helps us extract more detailed environmental features and improve the resolution and accuracy of environmental data.
It also helps researchers identify key health predictors and make reliable predictions over time. With GeoAI, researchers can reconstruct past exposures and understand how they affect health outcomes.
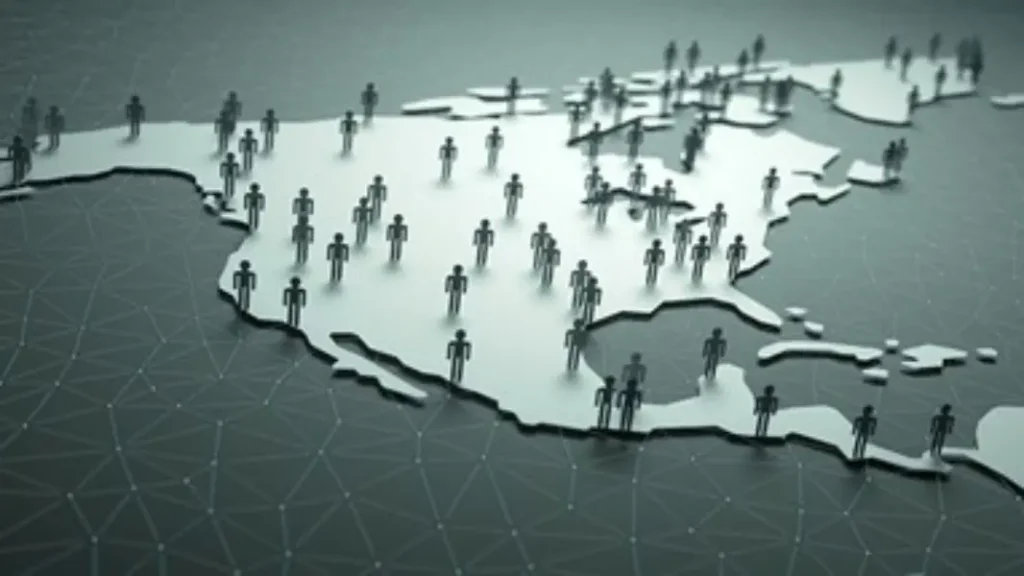
By combining GeoAI with traditional methods, researchers can overcome the limitations of these methods. As a result, they can gain a more dynamic and comprehensive understanding of how environmental factors influence health differences at different stages of life. This integration can help fill gaps in current epidemiological research by providing more accurate and efficient tools for analysing spatial and temporal health data.
Also read: OpenAI goes ‘Strawberry’, a new reasoning technology
Pop Quiz
Which technology is revolutionising the way we analyse spatial data to improve public health and environmental management?
A) Blockchain
B) Internet of Things (IoT)
C) Cloud computing
D) GeoAI
The correct answer is at the bottom of the article.
GeoAI applications for health and healthcare
In Oakland, California, for example, air pollution is a major concern. To tackle the problem, Google Street View cars were fitted with air pollution sensors and driven around the city to map air quality in detail. This GeoAI project turned every street into a data point, providing a clear picture of where the air was most and least polluted. The data collected became a powerful tool for local government, and allowed them to make informed decisions about environmental policy and public health interventions.
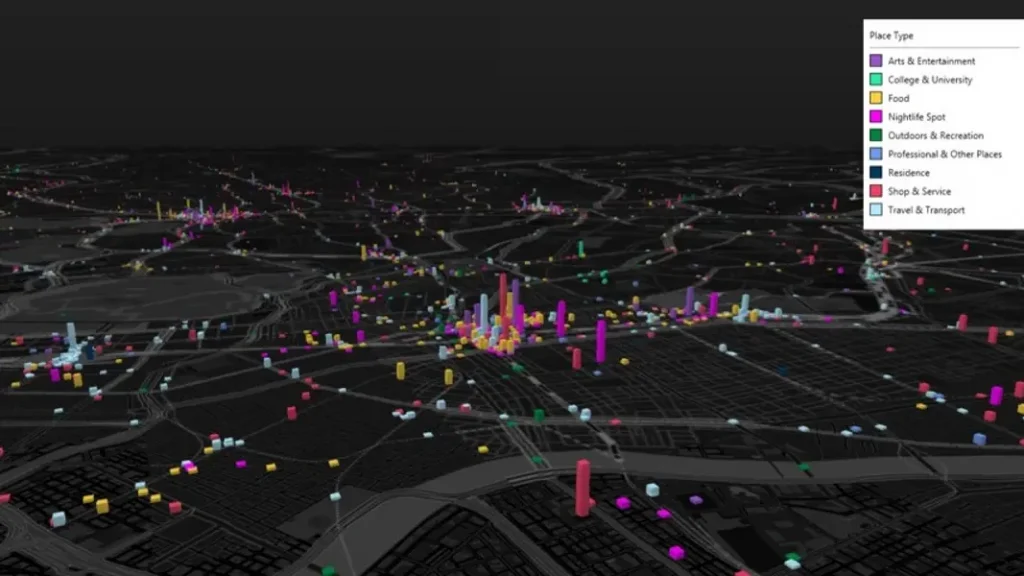
What’s more, the fight against flu in the US is being transformed by GeoAI. Traditional surveillance methods often can’t keep up with the virus. Now, using lots of data from sources like Google Flu Trends and climate reports, GeoAI models using deep learning algorithms predict where and when flu will hit hardest. This helps healthcare providers prepare more effectively, ensuring resources like vaccines are distributed efficiently and public health responses are timely and effective.
GeoAI in mining
While GeoAI significantly improves our understanding of environmental impacts on public health in spatial epidemiology by helping to identify the complex relationships between environmental conditions and health outcomes. It also plays a critical role in promoting environmental responsibility in the mining sector.
GeoAI, or Geospatial Artificial Intelligence, is revolutionising the mining industry by improving efficiency and sustainability. This technology uses AI and geospatial data to optimise mining operations from planning to execution.
For example, GeoAI enables the analysis of satellite imagery and other geospatial data to identify potential mineral deposits. By identifying geological features visible in satellite imagery – such as rock formations, fault lines and soil types – and historical mining data, GeoAI can predict the location of valuable minerals such as gold, reducing the need for extensive ground exploration.
Geospatial AI tools could enable mining companies and regulators to effectively monitor mining activities.
Kabo-Bah, associate professor at the University of Energy and Natural Resource
Integrating GeoAI into mining operations not only streamlines the exploration phase, but also minimises environmental impact. Accurate predictions of mineral locations allow for more focused mining plans, reducing ground disturbance and waste, and helping companies meet their sustainability goals.
In particular, GeoAI’s role in improving operational efficiency and environmental protection is underscored by initiatives such as Ghana’s National Geospatial Policy, which aims to regulate natural resources and establish the country as a leading mining hub in Africa.
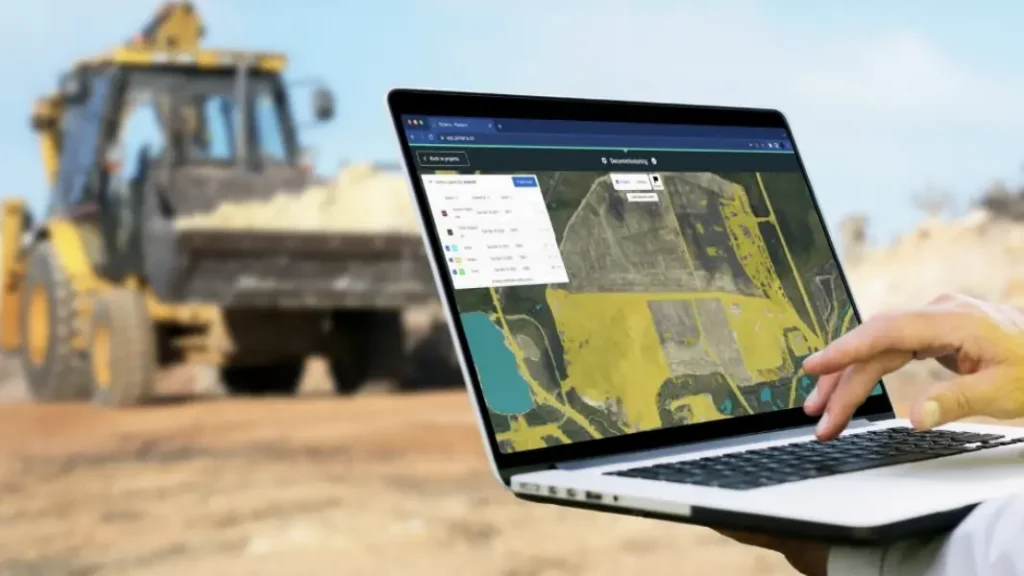
GeoAI is also significantly improving transparency in the mining sector. Tools powered by GeoAI enable effective monitoring of mining activities, ensuring that operations, regardless of size, comply with regulatory standards.
This makes the technology critical in regions such as Ghana, where mining has a significant impact on water resources and land reclamation is critical. By providing real-time monitoring and data analysis, GeoAI helps enforce compliance and safety standards, preventing accidents and ensuring worker safety.
What’s more, the proactive use of GeoAI goes far beyond compliance. It’s also about protecting the environment. Kabo-Bah, an associate professor at the University of Energy and Natural Resources, stressed the importance of maintaining the value of land after mining.
He emphasised the need to preserve the value of land for sustainable livelihoods and suggested that geospatial AI could provide critical data and insights for effective land use management, thereby supporting sustainable practices.
In addition, GeoAI is really useful for keeping an eye on illegal mining, especially in Ghana where it is a big problem. It helps to identify unregulated mining sites and assess their impact on the environment and local communities. This is important in ensuring that mining operations are responsible and follow environmental standards.
Overall, GeoAI is more than just a technological improvement. It’s about making practices more responsible, sustainable and efficient. It’s a big step towards environmental responsibility and shows that the industry recognises the need for sustainable mining solutions.
Also read: The powerful synergy of big data and AI: Transforming our world
New opportunities and challenges
The new field of GeoAI offers some great opportunities to develop spatial epidemiology. It allows us to look at how different levels and stages of life affect health, which helps us to understand the different ways in which the environment affects our health.
However, there are some challenges to implementing GeoAI. One major challenge is that we need large, well-labelled datasets to train accurate and effective AI models. Collecting and preparing these datasets is often laborious and time-consuming, making it difficult to rapidly deploy new GeoAI solutions in research.
By overcoming these challenges and making GeoAI a bigger part of spatial epidemiology, researchers can improve their methods and help create better public health strategies and policies. This change is a major step forward in our ability to use new technologies to understand and tackle health inequalities.
GeoAI also faces challenges in integrating with different systems. A major issue is the high demand for data quality and the difficulty of interfacing with legacy systems. There’s also a critical need for skilled personnel capable of managing and developing GeoAI technologies.
Potential solutions include improving AI training methods, improving data collection and processing techniques, and fostering collaboration between technology developers and industry experts to tailor GeoAI solutions to specific needs.
Future outlook of GeoAI
Looking to the future, the potential of GeoAI is huge, with expected advances in AI and machine learning that will further push the boundaries of spatial data analysis. These developments could significantly improve predictive capabilities for environmental impact and resource management.
Most importantly, the evolution of GeoAI could also have a profound impact on public health strategies by providing more accurate data analysis, and similarly transform the resource extraction industry by optimising processes and increasing sustainability.
Correct answer: D. GeoAI.